馃摎 This guide explains how to use **Weights & Biases** (W&B) with YOLOv5 馃殌. UPDATED 29 September 2021.
* [About Weights & Biases](#about-weights-&-biases)
* [First-Time Setup](#first-time-setup)
* [Viewing runs](#viewing-runs)
* [Advanced Usage: Dataset Versioning and Evaluation](#advanced-usage)
* [Reports: Share your work with the world!](#reports)
## About Weights & Biases
Think of [W&B](https://wandb.ai/site?utm_campaign=repo_yolo_wandbtutorial) like GitHub for machine learning models. With a few lines of code, save everything you need to debug, compare and reproduce your models 鈥� architecture, hyperparameters, git commits, model weights, GPU usage, and even datasets and predictions.
Used by top researchers including teams at OpenAI, Lyft, Github, and MILA, W&B is part of the new standard of best practices for machine learning. How W&B can help you optimize your machine learning workflows:
* [Debug](https://wandb.ai/wandb/getting-started/reports/Visualize-Debug-Machine-Learning-Models--VmlldzoyNzY5MDk#Free-2) model performance in real time
* [GPU usage](https://wandb.ai/wandb/getting-started/reports/Visualize-Debug-Machine-Learning-Models--VmlldzoyNzY5MDk#System-4) visualized automatically
* [Custom charts](https://wandb.ai/wandb/customizable-charts/reports/Powerful-Custom-Charts-To-Debug-Model-Peformance--VmlldzoyNzY4ODI) for powerful, extensible visualization
* [Share insights](https://wandb.ai/wandb/getting-started/reports/Visualize-Debug-Machine-Learning-Models--VmlldzoyNzY5MDk#Share-8) interactively with collaborators
* [Optimize hyperparameters](https://docs.wandb.com/sweeps) efficiently
* [Track](https://docs.wandb.com/artifacts) datasets, pipelines, and production models
## First-Time Setup
<details open>
<summary> Toggle Details </summary>
When you first train, W&B will prompt you to create a new account and will generate an **API key** for you. If you are an existing user you can retrieve your key from https://wandb.ai/authorize. This key is used to tell W&B where to log your data. You only need to supply your key once, and then it is remembered on the same device.
W&B will create a cloud **project** (default is 'YOLOv5') for your training runs, and each new training run will be provided a unique run **name** within that project as project/name. You can also manually set your project and run name as:
```shell
$ python train.py --project ... --name ...
```
YOLOv5 notebook example: <a href="https://colab.research.google.com/github/ultralytics/yolov5/blob/master/tutorial.ipynb"><img src="https://colab.research.google.com/assets/colab-badge.svg" alt="Open In Colab"></a> <a href="https://www.kaggle.com/ultralytics/yolov5"><img src="https://kaggle.com/static/images/open-in-kaggle.svg" alt="Open In Kaggle"></a>
<img width="960" alt="Screen Shot 2021-09-29 at 10 23 13 PM" src="https://user-images.githubusercontent.com/26833433/135392431-1ab7920a-c49d-450a-b0b0-0c86ec86100e.png">
</details>
## Viewing Runs
<details open>
<summary> Toggle Details </summary>
Run information streams from your environment to the W&B cloud console as you train. This allows you to monitor and even cancel runs in <b>realtime</b> . All important information is logged:
* Training & Validation losses
* Metrics: Precision, Recall, mAP@0.5, mAP@0.5:0.95
* Learning Rate over time
* A bounding box debugging panel, showing the training progress over time
* GPU: Type, **GPU Utilization**, power, temperature, **CUDA memory usage**
* System: Disk I/0, CPU utilization, RAM memory usage
* Your trained model as W&B Artifact
* Environment: OS and Python types, Git repository and state, **training command**
<p align="center"><img width="900" alt="Weights & Biases dashboard" src="https://user-images.githubusercontent.com/26833433/135390767-c28b050f-8455-4004-adb0-3b730386e2b2.png"></p>
</details>
## Advanced Usage
You can leverage W&B artifacts and Tables integration to easily visualize and manage your datasets, models and training evaluations. Here are some quick examples to get you started.
<details open>
<h3>1. Visualize and Version Datasets</h3>
Log, visualize, dynamically query, and understand your data with <a href='http://222.178.203.72:19005/whst/63/=cnbrzvZmcazZh//guides/data-vis/tables'>W&B Tables</a>. You can use the following command to log your dataset as a W&B Table. This will generate a <code>{dataset}_wandb.yaml</code> file which can be used to train from dataset artifact.
<details>
<summary> <b>Usage</b> </summary>
<b>Code</b> <code> $ python utils/logger/wandb/log_dataset.py --project ... --name ... --data .. </code>
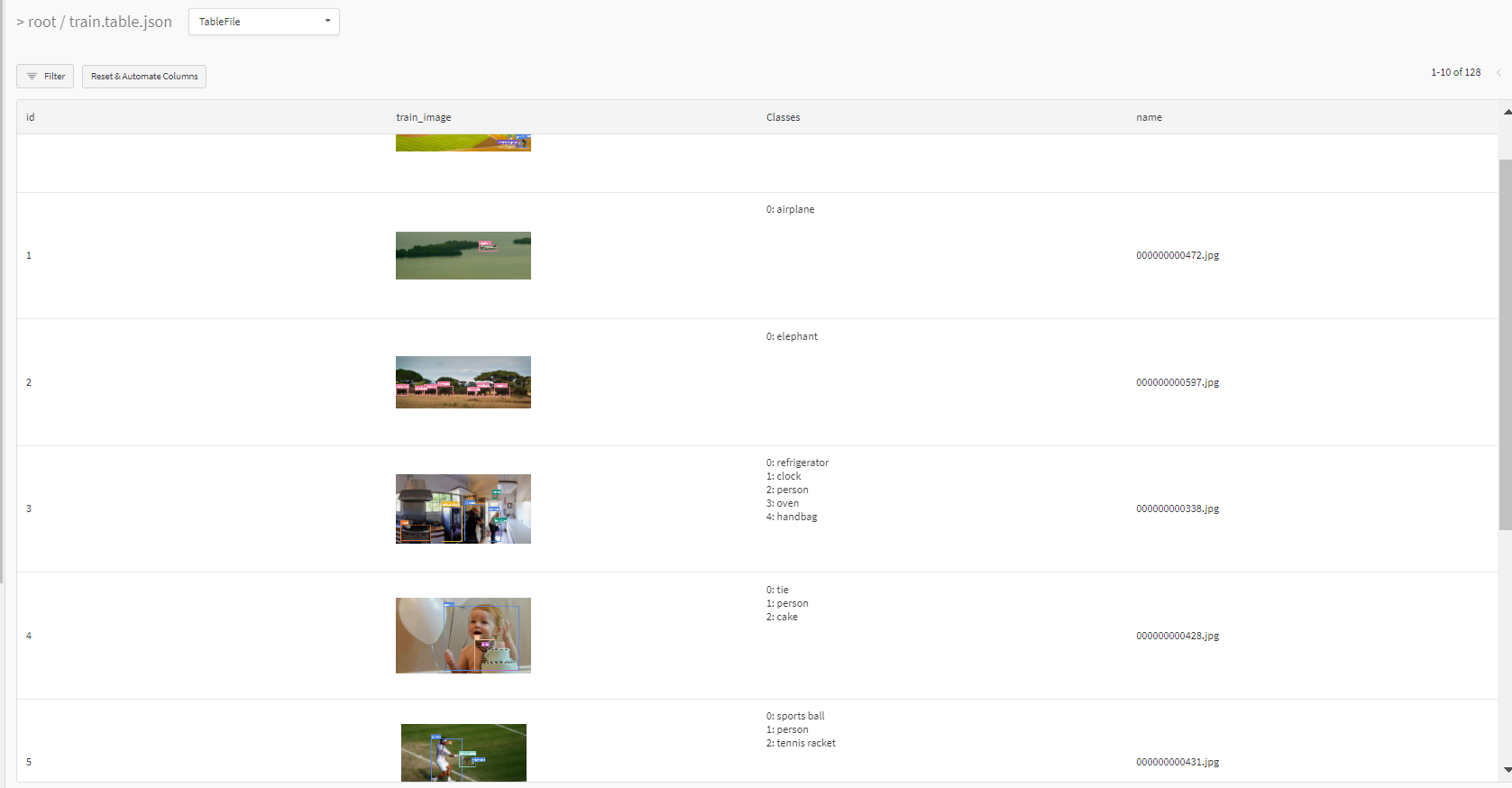
</details>
<h3> 2: Train and Log Evaluation simultaneousy </h3>
This is an extension of the previous section, but it'll also training after uploading the dataset. <b> This also evaluation Table</b>
Evaluation table compares your predictions and ground truths across the validation set for each epoch. It uses the references to the already uploaded datasets,
so no images will be uploaded from your system more than once.
<details>
<summary> <b>Usage</b> </summary>
<b>Code</b> <code> $ python utils/logger/wandb/log_dataset.py --data .. --upload_data </code>
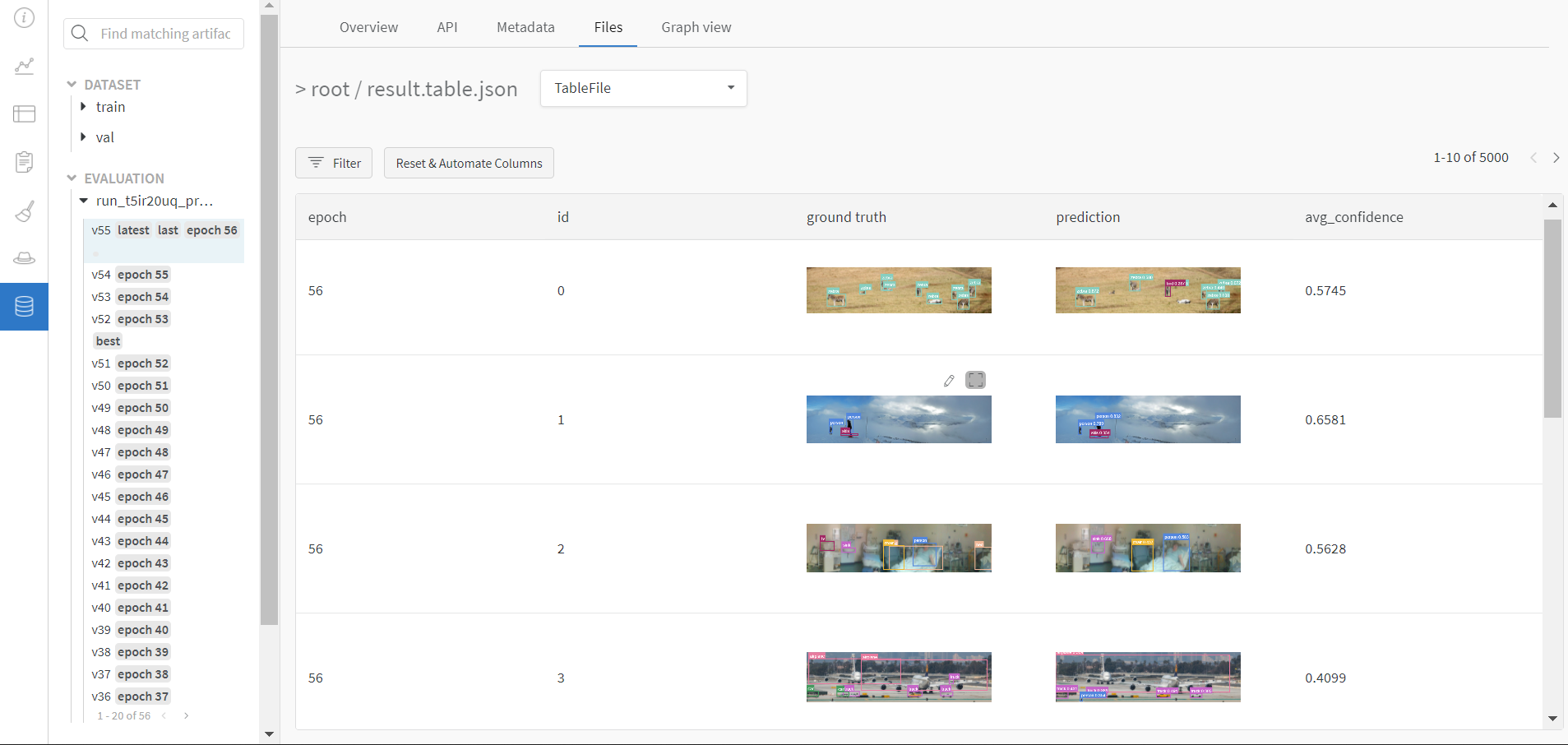
</details>
<h3> 3: Train using dataset artifact </h3>
When you upload a dataset as described in the first section, you get a new config file with an added `_wandb` to its name. This file contains the information that
can be used to train a model directly from the dataset artifact. <b> This also logs evaluation </b>
<details>
<summary> <b>Usage</b> </summary>
<b>Code</b> <code> $ python utils/logger/wandb/log_dataset.py --data {data}_wandb.yaml </code>
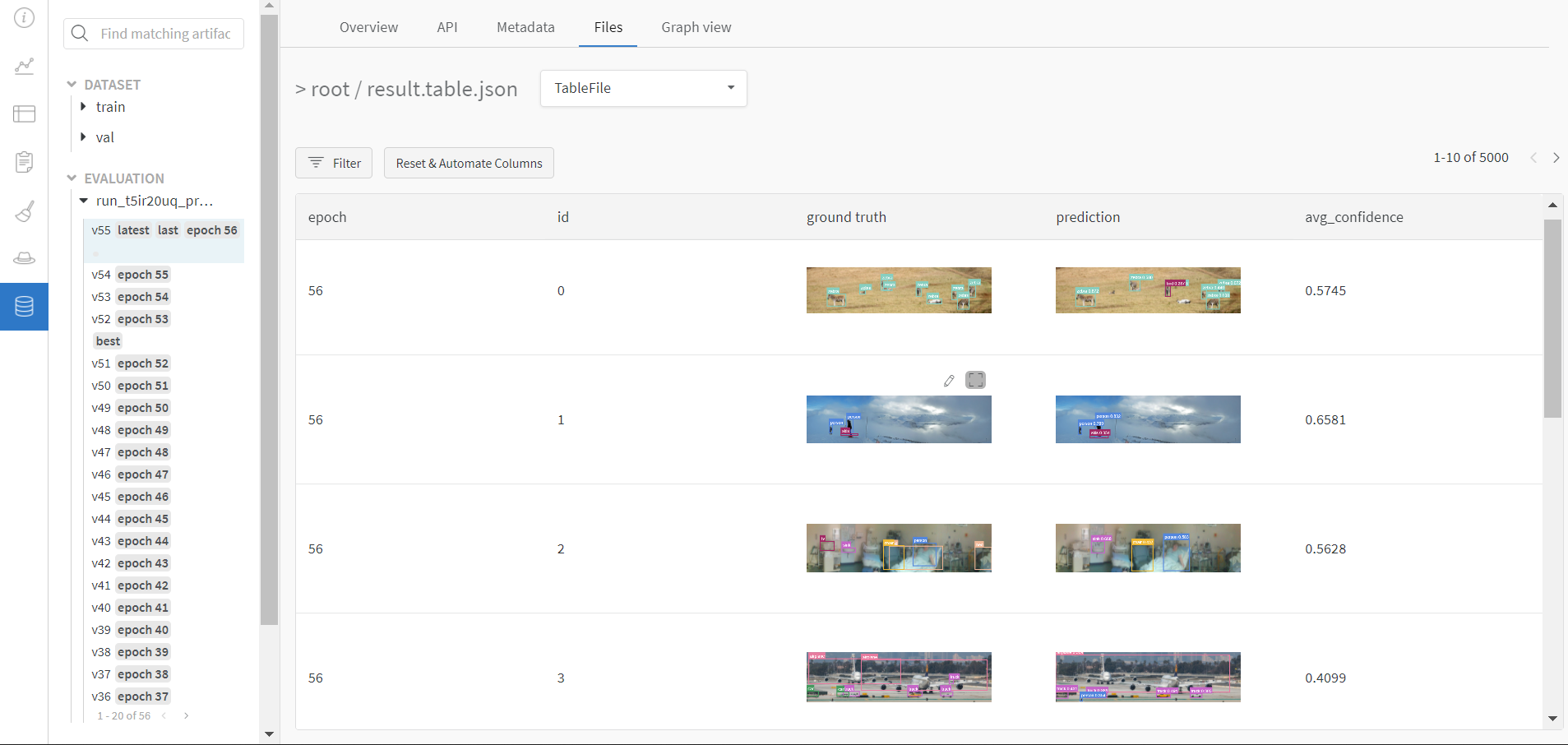
</details>
<h3> 4: Save model checkpoints as artifacts </h3>
To enable saving and versioning checkpoints of your experiment, pass `--save_period n` with the base cammand, where `n` represents checkpoint interval.
You can also log both the dataset and model checkpoints simultaneously. If not passed, only the final model will be logged
<details>
<summary> <b>Usage</b> </summary>
<b>Code</b> <code> $ python train.py --save_period 1 </code>
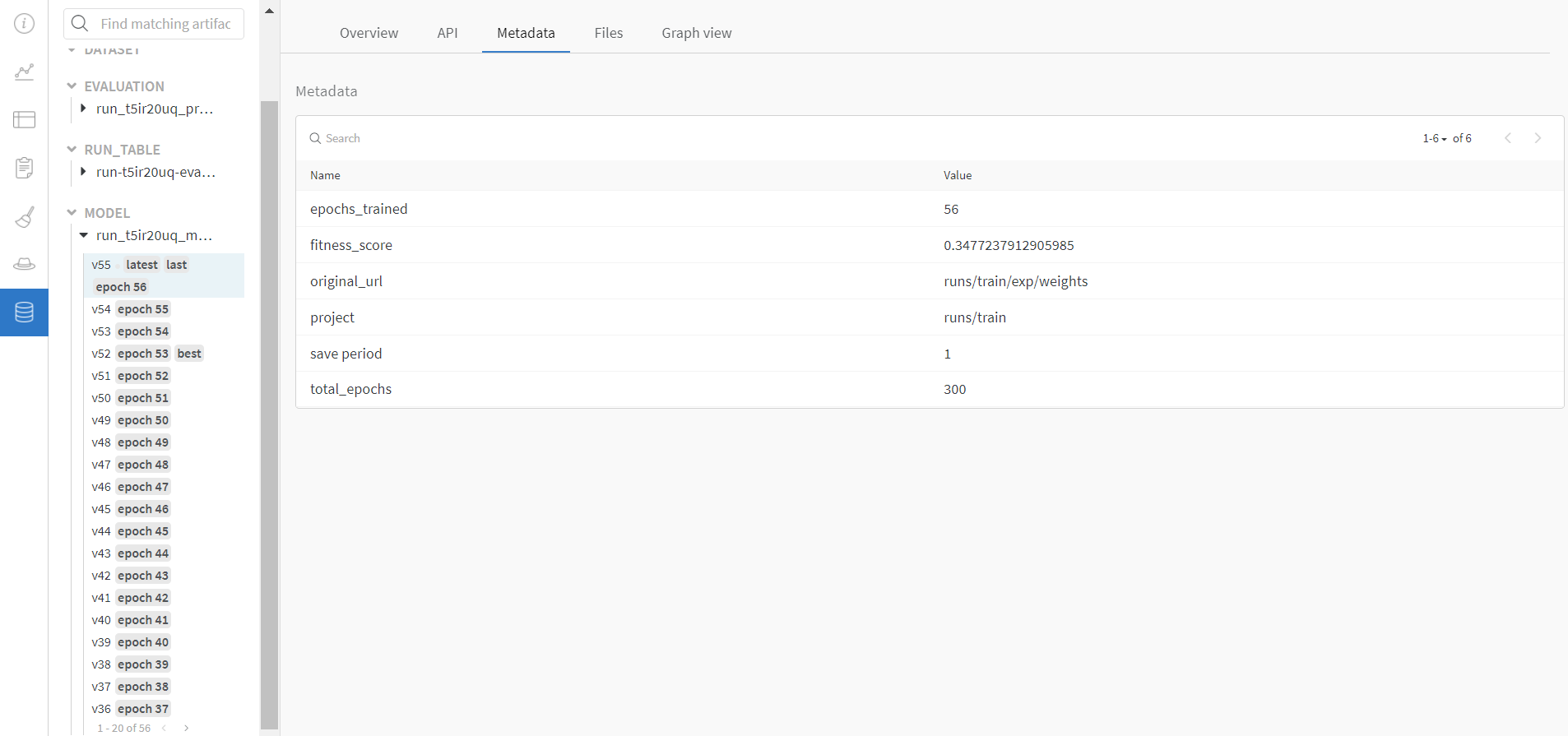
</details>
</details>
<h3> 5: Resume runs from checkpoint artifacts. </h3>
Any run can be resumed using artifacts if the <code>--resume</code> argument starts with聽<code>wandb-artifact://</code>聽prefix followed by the run path, i.e,聽<code>wandb-artifact://username/project/runid </code>. This doesn't require the model checkpoint to be present on the local system.
<details>
<summary> <b>Usage</b> </summary>
<b>Code</b> <code> $ python train.py --resume wandb-artifact://{run_path} </code>
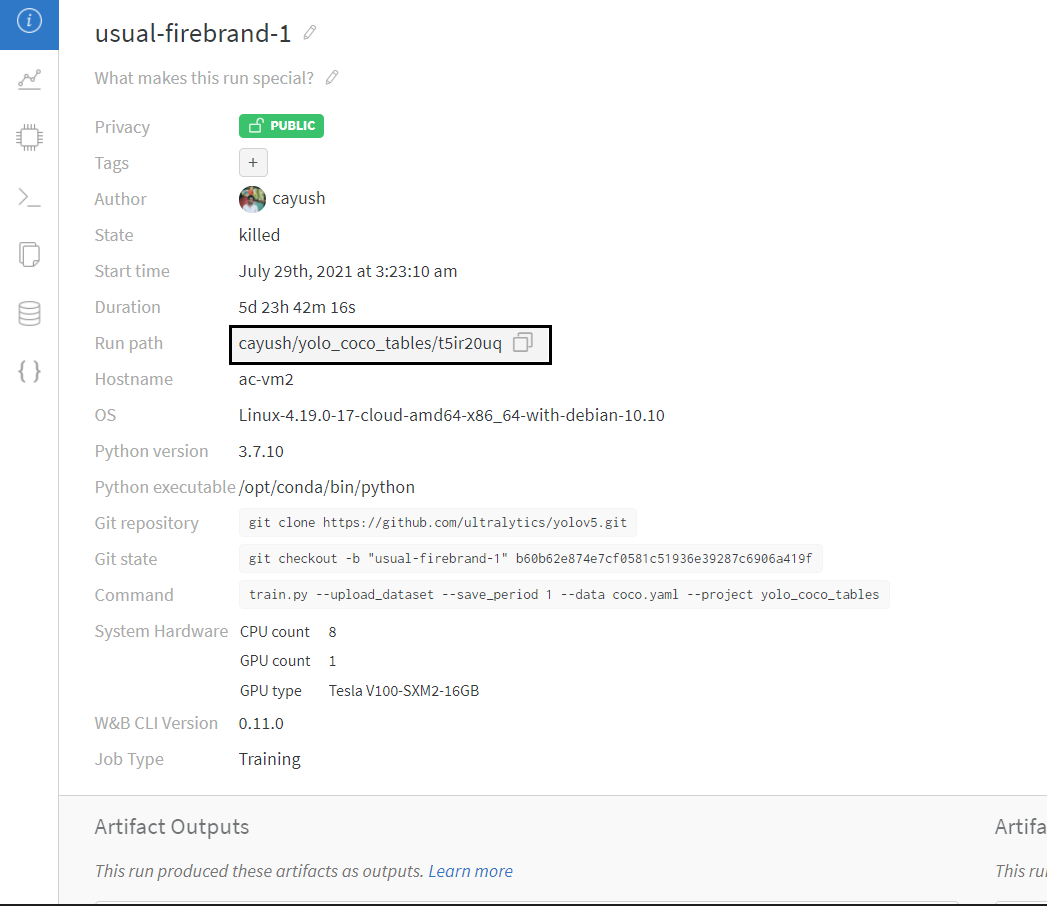
</details>
<h3> 6: Resume runs from dataset artifact & checkpoint artifacts. </h3>
<b> Local dataset or model checkpoints are not required. This can be used to resume runs directly on a different device </b>
The syntax is same as the previous section, but you'll need to lof both the dataset and model checkpoints as artifacts, i.e, set bot <code>--upload_dataset</code> or
train from <code>_wandb.yaml</code> file and set <code>--save_period</code>
<details>
<summary> <b>Usage</b> </summary>
<b>Code</b> <code> $ python train.py --resume wandb-artifact://{run_path} </code>
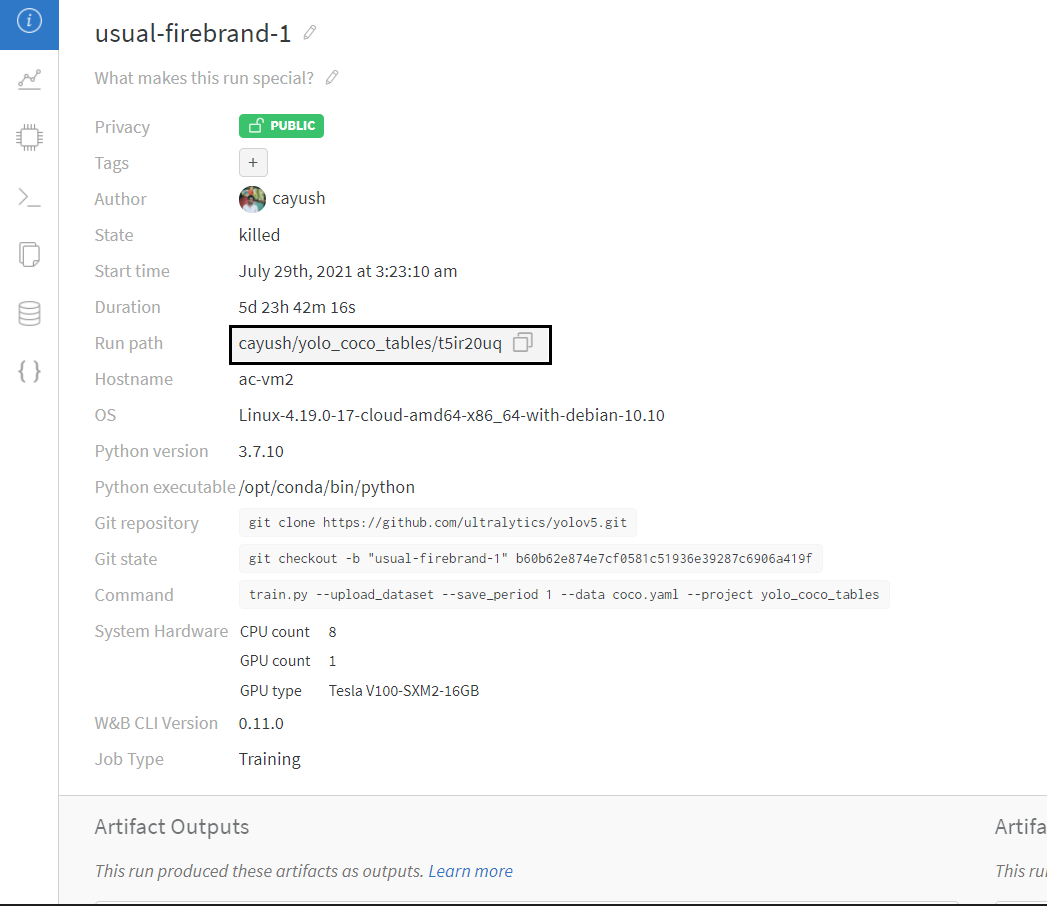
</details>
</details>

onnx
- 粉丝: 1w+
- 资源: 5637
最新资源
- matlab实现四旋翼无人机自抗扰姿态容错控制-飞行器控制-四旋翼无人机-自抗扰控制-UAV-扰动识别-matlab
- 基于主从博弈的综合能源微网与共享储能优化运行研究:迭代算法探索与利益相关者的博弈策略,基于主从博弈的综合能源微网与共享储能优化运行研究:电价策略与用户行为互动,matlab 代码基于主从博弈的共享储能
- 14章RAG全栈技术从基础到精通 ,打造高精准AI应用
- MATLAB平台下的多目标粒子群算法在冷热电联供综合能源系统优化中的实践,基于多目标粒子群算法的冷热电联供综合能源系统优化调度模型,MATLAB代码:基于多目标粒子群算法冷热电联供综合能源系统运行优化
- 三相桥式全控整流及其有源逆变与Simulink仿真波形分析(含不同触发角和负载变化图),三相桥式全控整流及其有源逆变与Simulink仿真探究:触发角与负载变化下的波形图分析,三相桥式全控整流及其有源
- 中国智能制造装备产业发展机遇蓝皮书 2024.pdf
- 【毕业设计】Python的Django-html网易新闻+评论的舆情热点分析平台源码(完整前后端+mysql+说明文档+LW+PPT).zip
- 西门子1200 PLC与昆仑通态上位软件结合的新能源物料输送系统程序案例解析,V16+博图版本,RS485通讯控制托利多称重仪表模拟量读取技术实现,西门子S1200博图物料输送系统:新能源物料输送PL
- 【毕业设计】Python的Django-html图像的信息隐藏技术研究源码(完整前后端+mysql+说明文档+LW+PPT).zip
- 【毕业设计】Python的Django-html深度学习的车牌识别系统源码(完整前后端+mysql+说明文档+LW+PPT).zip
- 【毕业设计】Python的Django-html深度学习屋内烟雾检测方法源码(完整前后端+mysql+说明文档+LW+PPT).zip
- 灰狼算法在微电网优化调度中的应用:考虑风光储与需求响应的多元经济优化策略,模型可塑性强且出图丰富的实践研究,灰狼算法在微电网风光储需求响应优化调度中的应用:经济性优化与模型可塑性的研究分析图集,灰狼算
- 【毕业设计】Python的Django-html人脸识别的实验室智能门禁系统源码(完整前后端+mysql+说明文档+LW+PPT).zip
- 【毕业设计】Python的Django-html深度学习的身份证识别考勤系统源码(完整前后端+mysql+说明文档+LW+PPT).zip
- 【毕业设计】Python的Django-html时间序列分析的大气污染预测系统源码(完整前后端+mysql+说明文档+LW+PPT).zip
- matlab实现遗传算法在物流中心配送路径规划中的应用-遗传算法-路径规划-物流配送-最佳配送方案-matlab
资源上传下载、课程学习等过程中有任何疑问或建议,欢迎提出宝贵意见哦~我们会及时处理!
点击此处反馈


