[](https://zenodo.org/badge/latestdoi/89872749)
# CNNGestureRecognizer
Gesture recognition via CNN neural network implemented in Keras + Theano + OpenCV
Key Requirements:
Python 2.7.13
OpenCV 2.4.8
Keras 2.0.2
Theano 0.9.0
Suggestion: Better to download Anaconda as it will take care of most of the other packages and easier to setup a virtual workspace to work with multiple versions of key packages like python, opencv etc.
# Repo contents
- **trackgesture.py** : The main script launcher. This file contains all the code for UI options and OpenCV code to capture camera contents. This script internally calls interfaces to gestureCNN.py.
- **gestureCNN.py** : This script file holds all the CNN specific code to create CNN model, load the weight file (if model is pretrained), train the model using image samples present in **./imgfolder_b**, visualize the feature maps at different layers of NN (of pretrained model) for a given input image present in **./imgs** folder.
- **imgfolder_b** : This folder contains all the 4015 gesture images I took in order to train the model.
- **_ori_4015imgs_weights.hdf5_** : This is pretrained file. If for some reason you find issues with downloading from github then it can be downloaded from my google driver link - https://drive.google.com/open?id=0B6cMRAuImU69SHNCcXpkT3RpYkE
- **_imgs_** - This is an optional folder of few sample images that one can use to visualize the feature maps at different layers. These are few sample images from imgfolder_b only.
- **_ori_4015imgs_acc.png_** : This is just a pic of a plot depicting model accuracy Vs validation data accuracy after I trained it.
- **_ori_4015imgs_loss.png_** : This is just a pic of a plot depicting model loss Vs validation loss after I training.
# Usage
```bash
$ KERAS_BACKEND=theano python trackgesture.py
```
We are setting KERAS_BACKEND to change backend to Theano, so in case you have already done it via Keras.json then no need to do that. But if you have Tensorflow set as default then this will be required.
# Features
This application comes with CNN model to recognize upto 5 pretrained gestures:
- OK
- PEACE
- STOP
- PUNCH
- NOTHING (ie when none of the above gestures are input)
This application provides following functionalities:
- Prediction : Which allows the app to guess the user's gesture against pretrained gestures. App can dump the prediction data to the console terminal or to a json file directly which can be used to plot real time prediction bar chart (you can use my other script - https://github.com/asingh33/LivePlot)
- New Training : Which allows the user to retrain the NN model. User can change the model architecture or add/remove new gestures. This app has inbuilt options to allow the user to create new image samples of user defined gestures if required.
- Visualization : Which allows the user to see feature maps of different NN layers for a given input gesture image. Interesting to see how NN works and learns things.
# Demo
Youtube link - https://www.youtube.com/watch?v=CMs5cn65YK8
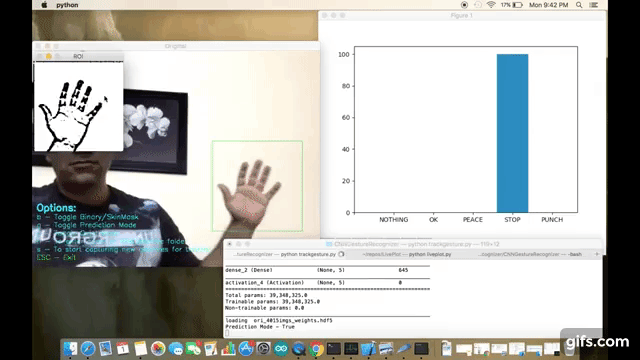
# Gesture Input
I am using OpenCV for capturing the user's hand gestures. In order to simply things I am doing post processing on the captured images to highlight the contours & edges. Like applying binary threshold, blurring, gray scaling.
I have provided two modes of capturing:
- Binary Mode : In here I first convert the image to grayscale, then apply a gaussian blur effect with adaptive threshold filter. This mode is useful when you have an empty background like a wall, whiteboard etc.
- SkinMask Mode : In this mode, I first convert the input image to HSV and put range on the H,S,V values based on skin color range. Then apply errosion followed by dilation. Then gaussian blur to smoothen out the noises. Using this output as a mask on original input to mask out everything other than skin colored things. Finally I have grayscaled it. This mode is useful when there is good amount of light and you dont have empty background.
**Binary Mode processing**
```python
gray = cv2.cvtColor(roi, cv2.COLOR_BGR2GRAY)
blur = cv2.GaussianBlur(gray,(5,5),2)
th3 = cv2.adaptiveThreshold(blur,255,cv2.ADAPTIVE_THRESH_GAUSSIAN_C,cv2.THRESH_BINARY_INV,11,2)
ret, res = cv2.threshold(th3, minValue, 255, cv2.THRESH_BINARY_INV+cv2.THRESH_OTSU)
```

**SkindMask Mode processing**
```python
hsv = cv2.cvtColor(roi, cv2.COLOR_BGR2HSV)
#Apply skin color range
mask = cv2.inRange(hsv, low_range, upper_range)
mask = cv2.erode(mask, skinkernel, iterations = 1)
mask = cv2.dilate(mask, skinkernel, iterations = 1)
#blur
mask = cv2.GaussianBlur(mask, (15,15), 1)
#cv2.imshow("Blur", mask)
#bitwise and mask original frame
res = cv2.bitwise_and(roi, roi, mask = mask)
# color to grayscale
res = cv2.cvtColor(res, cv2.COLOR_BGR2GRAY)
```

# CNN Model used
The CNN I have used for this project is pretty common CNN model which can be found across various tutorials on CNN. Mostly I have seen it being used for Digit/Number classfication based on MNIST database.
```python
model = Sequential()
model.add(Conv2D(nb_filters, (nb_conv, nb_conv),
padding='valid',
input_shape=(img_channels, img_rows, img_cols)))
convout1 = Activation('relu')
model.add(convout1)
model.add(Conv2D(nb_filters, (nb_conv, nb_conv)))
convout2 = Activation('relu')
model.add(convout2)
model.add(MaxPooling2D(pool_size=(nb_pool, nb_pool)))
model.add(Dropout(0.5))
model.add(Flatten())
model.add(Dense(128))
model.add(Activation('relu'))
model.add(Dropout(0.5))
model.add(Dense(nb_classes))
model.add(Activation('softmax'))
```
This model has following 12 layers -
```
_________________________________________________________________
Layer (type) Output Shape Param #
=================================================================
conv2d_1 (Conv2D) (None, 32, 198, 198) 320
_________________________________________________________________
activation_1 (Activation) (None, 32, 198, 198) 0
_________________________________________________________________
conv2d_2 (Conv2D) (None, 32, 196, 196) 9248
_________________________________________________________________
activation_2 (Activation) (None, 32, 196, 196) 0
_________________________________________________________________
max_pooling2d_1 (MaxPooling2 (None, 32, 98, 98) 0
_________________________________________________________________
dropout_1 (Dropout) (None, 32, 98, 98) 0
_________________________________________________________________
flatten_1 (Flatten) (None, 307328) 0
_________________________________________________________________
dense_1 (Dense) (None, 128) 39338112
_________________________________________________________________
activation_3 (Activation) (None, 128) 0
_________________________________________________________________
dropout_2 (Dropout) (None, 128) 0
_________________________________________________________________
dense_2 (Dense) (None, 5) 645
_________________________________________________________________
activation_4 (Activation) (None, 5) 0
=================================================================
```
Total params: 39,348,325.0
Trainable params: 39,348,325.0
# Training
In version 1.0 of this project I had used 1204 images only for training. Predictions probability was ok but not satisfying. So in version 2.0 I increased the training image set to 4015 images i.e. 803 ima
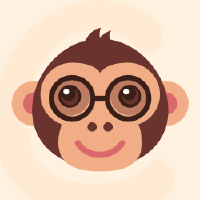
liu1823612484
- 粉丝: 2
- 资源: 16
最新资源
- 基于java+ssm+mysql+微信小程序的插画共享平台 源码+数据库+论文(高分毕设项目).zip
- 基于java+ssm+mysql+微信小程序的车视界小程序 源码+数据库+论文(高分毕设项目).zip
- 基于java+ssm+mysql+微信小程序的场地预约系统 源码+数据库+论文(高分毕设项目).zip
- 基于java+ssm+mysql+微信小程序的宠物店商城小程序 源码+数据库+论文(高分毕设项目).zip
- 基于java+ssm+mysql+微信小程序的答题小程序 源码+数据库+论文(高分毕设项目).zip
- 基于java+ssm+mysql+微信小程序的村游网站系统 源码+数据库+论文(高分毕设项目).zip
- 欧姆龙NJ NX系列利用POD映射扩展轴功能块与应用案例:多轴控制拓展至更高轴数(超越传统限制),欧姆龙NJ NX使用POD映射拓展轴功能块与应用案例,可以在原有轴数(8.16.32.64)基础上实现
- 基于java+ssm+mysql+微信小程序的宠物寄养平台 源码+数据库+论文(高分毕设项目).zip
- 基于java+ssm+mysql+微信小程序的打印室预约系统 源码+数据库+论文(高分毕设项目).zip
- 基于java+ssm+mysql+微信小程序的大学生社团活动管理系统 源码+数据库+论文(高分毕设项目).zip
- ESP32在Espressif-IDE中集成与使用Wokwi仿真技术详解
- 基于java+ssm+mysql+微信小程序的点餐系统 源码+数据库+论文(高分毕设项目).zip
- 基于Matlab Simulink的空气悬架模块化非线性建模:精细化仿真,整车动力学学习的好帮手,空气悬架建模 软件使用:Matlab Simulink 适用场景:采用模块化建模方法,搭建非线性空气悬
- 基于java+ssm+mysql+微信小程序的高校党费收缴系统 源码+数据库+论文(高分毕设项目).zip
- C# Windows窗体图书管理系统:远程操作,含文档,实现数据库增删查改与登录注册功能,支持图片上传,C#Windows窗体开发的图书管理系统,可远程,有文档,供学习参考使用,主要功能:涵盖数据库增
- 基于java+ssm+mysql+微信小程序的高校暑期社会实践小程序 源码+数据库+论文(高分毕设项目).zip
资源上传下载、课程学习等过程中有任何疑问或建议,欢迎提出宝贵意见哦~我们会及时处理!
点击此处反馈


