<img src="https://cdn.comet.ml/img/notebook_logo.png">
# YOLOv5 with Comet
This guide will cover how to use YOLOv5 with [Comet](https://www.comet.com/site/?ref=yolov5&utm_source=yolov5&utm_medium=affilliate&utm_campaign=yolov5_comet_integration)
# About Comet
Comet builds tools that help data scientists, engineers, and team leaders accelerate and optimize machine learning and deep learning models.
Track and visualize model metrics in real time, save your hyperparameters, datasets, and model checkpoints, and visualize your model predictions with [Comet Custom Panels](https://www.comet.com/examples/comet-example-yolov5?shareable=YcwMiJaZSXfcEXpGOHDD12vA1&ref=yolov5&utm_source=yolov5&utm_medium=affilliate&utm_campaign=yolov5_comet_integration)!
Comet makes sure you never lose track of your work and makes it easy to share results and collaborate across teams of all sizes!
# Getting Started
## Install Comet
```shell
pip install comet_ml
```
## Configure Comet Credentials
There are two ways to configure Comet with YOLOv5.
You can either set your credentials through enviroment variables
**Environment Variables**
```shell
export COMET_API_KEY=<Your Comet API Key>
export COMET_PROJECT_NAME=<Your Comet Project Name> # This will default to 'yolov5'
```
Or create a `.comet.config` file in your working directory and set your credentials there.
**Comet Configuration File**
```
[comet]
api_key=<Your Comet API Key>
project_name=<Your Comet Project Name> # This will default to 'yolov5'
```
## Run the Training Script
```shell
# Train YOLOv5s on COCO128 for 5 epochs
python train.py --img 640 --batch 16 --epochs 5 --data coco128.yaml --weights yolov5s.pt
```
That's it! Comet will automatically log your hyperparameters, command line arguments, training and valiation metrics. You can visualize and analyze your runs in the Comet UI
<img width="1920" alt="yolo-ui" src="https://user-images.githubusercontent.com/7529846/187608607-ff89c3d5-1b8b-4743-a974-9275301b0524.png">
# Try out an Example!
Check out an example of a [completed run here](https://www.comet.com/examples/comet-example-yolov5/a0e29e0e9b984e4a822db2a62d0cb357?experiment-tab=chart&showOutliers=true&smoothing=0&transformY=smoothing&xAxis=step&ref=yolov5&utm_source=yolov5&utm_medium=affilliate&utm_campaign=yolov5_comet_integration)
Or better yet, try it out yourself in this Colab Notebook
[](https://colab.research.google.com/drive/1RG0WOQyxlDlo5Km8GogJpIEJlg_5lyYO?usp=sharing)
# Log automatically
By default, Comet will log the following items
## Metrics
- Box Loss, Object Loss, Classification Loss for the training and validation data
- mAP_0.5, mAP_0.5:0.95 metrics for the validation data.
- Precision and Recall for the validation data
## Parameters
- Model Hyperparameters
- All parameters passed through the command line options
## Visualizations
- Confusion Matrix of the model predictions on the validation data
- Plots for the PR and F1 curves across all classes
- Correlogram of the Class Labels
# Configure Comet Logging
Comet can be configured to log additional data either through command line flags passed to the training script
or through environment variables.
```shell
export COMET_MODE=online # Set whether to run Comet in 'online' or 'offline' mode. Defaults to online
export COMET_MODEL_NAME=<your model name> #Set the name for the saved model. Defaults to yolov5
export COMET_LOG_CONFUSION_MATRIX=false # Set to disable logging a Comet Confusion Matrix. Defaults to true
export COMET_MAX_IMAGE_UPLOADS=<number of allowed images to upload to Comet> # Controls how many total image predictions to log to Comet. Defaults to 100.
export COMET_LOG_PER_CLASS_METRICS=true # Set to log evaluation metrics for each detected class at the end of training. Defaults to false
export COMET_DEFAULT_CHECKPOINT_FILENAME=<your checkpoint filename> # Set this if you would like to resume training from a different checkpoint. Defaults to 'last.pt'
export COMET_LOG_BATCH_LEVEL_METRICS=true # Set this if you would like to log training metrics at the batch level. Defaults to false.
export COMET_LOG_PREDICTIONS=true # Set this to false to disable logging model predictions
```
## Logging Checkpoints with Comet
Logging Models to Comet is disabled by default. To enable it, pass the `save-period` argument to the training script. This will save the
logged checkpoints to Comet based on the interval value provided by `save-period`
```shell
python train.py \
--img 640 \
--batch 16 \
--epochs 5 \
--data coco128.yaml \
--weights yolov5s.pt \
--save-period 1
```
## Logging Model Predictions
By default, model predictions (images, ground truth labels and bounding boxes) will be logged to Comet.
You can control the frequency of logged predictions and the associated images by passing the `bbox_interval` command line argument. Predictions can be visualized using Comet's Object Detection Custom Panel. This frequency corresponds to every Nth batch of data per epoch. In the example below, we are logging every 2nd batch of data for each epoch.
**Note:** The YOLOv5 validation dataloader will default to a batch size of 32, so you will have to set the logging frequency accordingly.
Here is an [example project using the Panel](https://www.comet.com/examples/comet-example-yolov5?shareable=YcwMiJaZSXfcEXpGOHDD12vA1&ref=yolov5&utm_source=yolov5&utm_medium=affilliate&utm_campaign=yolov5_comet_integration)
```shell
python train.py \
--img 640 \
--batch 16 \
--epochs 5 \
--data coco128.yaml \
--weights yolov5s.pt \
--bbox_interval 2
```
### Controlling the number of Prediction Images logged to Comet
When logging predictions from YOLOv5, Comet will log the images associated with each set of predictions. By default a maximum of 100 validation images are logged. You can increase or decrease this number using the `COMET_MAX_IMAGE_UPLOADS` environment variable.
```shell
env COMET_MAX_IMAGE_UPLOADS=200 python train.py \
--img 640 \
--batch 16 \
--epochs 5 \
--data coco128.yaml \
--weights yolov5s.pt \
--bbox_interval 1
```
### Logging Class Level Metrics
Use the `COMET_LOG_PER_CLASS_METRICS` environment variable to log mAP, precision, recall, f1 for each class.
```shell
env COMET_LOG_PER_CLASS_METRICS=true python train.py \
--img 640 \
--batch 16 \
--epochs 5 \
--data coco128.yaml \
--weights yolov5s.pt
```
## Uploading a Dataset to Comet Artifacts
If you would like to store your data using [Comet Artifacts](https://www.comet.com/docs/v2/guides/data-management/using-artifacts/#learn-more?ref=yolov5&utm_source=yolov5&utm_medium=affilliate&utm_campaign=yolov5_comet_integration), you can do so using the `upload_dataset` flag.
The dataset be organized in the way described in the [YOLOv5 documentation](https://docs.ultralytics.com/tutorials/train-custom-datasets/#3-organize-directories). The dataset config `yaml` file must follow the same format as that of the `coco128.yaml` file.
```shell
python train.py \
--img 640 \
--batch 16 \
--epochs 5 \
--data coco128.yaml \
--weights yolov5s.pt \
--upload_dataset
```
You can find the uploaded dataset in the Artifacts tab in your Comet Workspace
<img width="1073" alt="artifact-1" src="https://user-images.githubusercontent.com/7529846/186929193-162718bf-ec7b-4eb9-8c3b-86b3763ef8ea.png">
You can preview the data directly in the Comet UI.
<img width="1082" alt="artifact-2" src="https://user-images.githubusercontent.com/7529846/186929215-432c36a9-c109-4eb0-944b-84c2786590d6.png">
Artifacts are versioned and also support adding metadata about the dataset. Comet will automatically log the metadata from your dataset `yaml` file
<img width="963" alt="artifact-3" src="https://user-images.githubusercontent.com/7529846/186929256-9d44d6eb-1a19-42de-889a-bcbca3018f2e.png">
### Using a saved Artifact
If you would like to use a dataset from Comet Artifacts, set the `path` variable in your dataset `yaml` file to point to the f
没有合适的资源?快使用搜索试试~ 我知道了~
温馨提示
基于yolov5实现打电话行为识别检测源码+训练好模型(毕业设计)含有代码注释,新手也可看懂,个人手打98分项目,毕业设计、期末大作业、课程设计、高分必看,下载下来,简单部署,就可以使用。该项目系统功能完善、界面美观、操作简单、功能齐全、管理便捷可以作为毕设、期末大作业使用。 基于yolov5实现打电话行为识别检测源码+训练好模型(毕业 基于yolov5实现打电话行为识别检测源码+训练好模型(毕业 基于yolov5实现打电话行为识别检测源码+训练好模型(毕业 基于yolov5实现打电话行为识别检测源码+训练好模型(毕业 基于yolov5实现打电话行为识别检测源码+训练好模型(毕业基于yolov5实现打电话行为识别检测源码+训练好模型(毕业基于yolov5实现打电话行为识别检测源码+训练好模型(毕业基于yolov5实现打电话行为识别检测源码+训练好模型(毕业基于yolov5实现打电话行为识别检测源码+训练好模型(毕业基于yolov5实现打电话行为识别检测源码+训练好模型(毕业基于yolov5实现打电话行为识别检测源码+训练好模型(毕业基于yolov5实现打电话行为识别检测源码+全部数
资源推荐
资源详情
资源评论
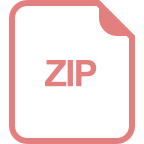
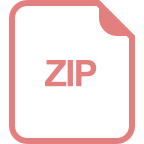
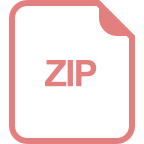
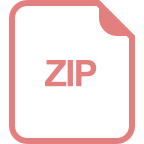
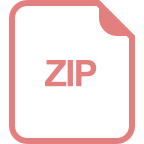
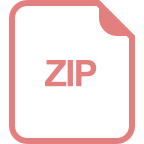
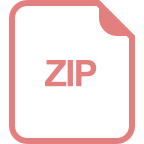
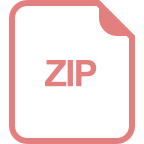
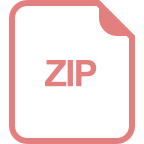
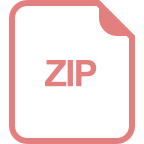
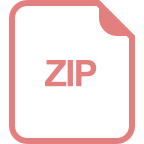
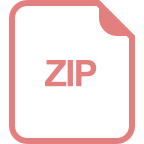
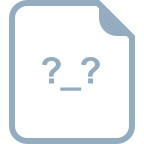
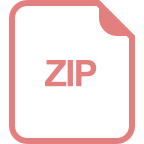
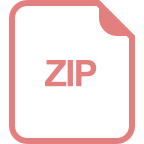
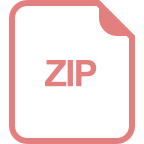
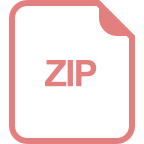
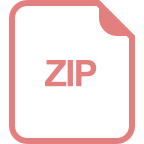
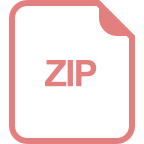
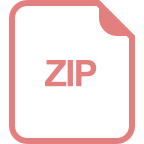
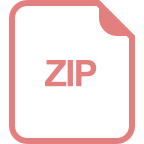
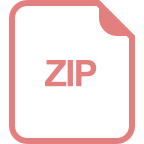
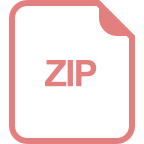
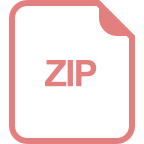
收起资源包目录





































































































共 142 条
- 1
- 2
资源评论


王二空间
- 粉丝: 7569
- 资源: 2110
上传资源 快速赚钱
我的内容管理 展开
我的资源 快来上传第一个资源
我的收益
登录查看自己的收益我的积分 登录查看自己的积分
我的C币 登录后查看C币余额
我的收藏
我的下载
下载帮助


最新资源
- 毕业设计A052-基于Java的健身房管理系统的设计与实现
- 模型预测电流控制-广义双矢量
- Python爬虫入门推荐+爬取商品数据进行数据分析+适用于测试、开发、运营等
- libusbK、libusb-win32、libwdi、USB视频设备 驱动安装包
- 江苏范特科技有限公司创投信息
- 上海零数科技有限公司创投信息
- 上海唯鲜良品食品科技有限公司创投信息
- 上海柚凡信息科技有限公司创投信息
- 上海域圆信息科技有限公司创投信息
- 上市公司财务指标数据集2023-2000年原始数据 含剔除金融STPT版本
- Qt中嵌入窗口,例如嵌入MainWindows、QWidget、QDialog等窗口
- matplotShowDataCSV2-最简单的数据绘图
- 深圳店匠科技有限公司创投信息
- 深圳莱芒生物科技有限公司创投信息
- 沈阳黛斯蓝伊莎生物科技有限公司创投信息
- 苏州引航生物科技有限公司创投信息
资源上传下载、课程学习等过程中有任何疑问或建议,欢迎提出宝贵意见哦~我们会及时处理!
点击此处反馈



安全验证
文档复制为VIP权益,开通VIP直接复制
