# 使用tensorflow构建卷积神经网络(CNN)
## **Convolutional Neural Networks (卷积神经网络)**
> **术语罗列**
- Convolutional layer 卷积层
- pooling layer 池化层
- Fully connected 全连接层
推荐阅读:[卷积神经网络工作原理直观的解释?](https://www.zhihu.com/question/39022858)
以下图片均来自于互联网,侵删。
## **简介**
1962年受Hubel和Wiesel对猫视觉皮层电生理研究启发,Kunihiko Fukishima(福岛邦彦)最先提出了卷积神经网络(CNN),近年来卷积神经网络发展迅速,在语音识别、人脸识别、通用物体识别、运动分析。tensorflow为手写字符推荐的算法就是卷积神经网络,模型识别率高达99.6%
## **卷积神经网络原理**
- 神经网络
- 含有隐含层的神经网络
- 卷积神经网络
- 使用python代码实现卷积神经网络
## **1.神经网络**
神经网络过程图
具体过程就是。神经信号**x**乘上权重向量**w**,经过输入函数(**Net input function**)求和后,由激活函数(**Activation function**)输出。监督学习过程中,输出结果将会对比数据集样本结果(**label**),使用损失函数(**cost function**)计算损失,并且经过优化器迭代后更新权重。
## **2.含有隐含层的神经网络**
神经网络隐含层
注意:Layer L1 为输入层,L2为隐含层,L3为输出层,h(x)为假设函数
这种神经网络叫做反向传播(**Back Propagation**)神经网络,简称BP网络,一般使用**sigmoid(s型)**激活函数。
## **3.卷积神经网络**
卷积神经网络包含了一个由卷积层和子采样层构成的特征抽取器。
卷积层通常包含若干个特征平面(**featureMap**),每一个特征平面由一些矩形排列的神经元组成,同一特征平面的神经元共享权值,这个共享权值就是卷积核。卷积核一般以随机小数矩阵的形式初始化,在网络的训练过程中卷积核将学习得到合理的权值。
子采样也叫做池化(**pooling**),通常有均值采样(mean poooling)和 最大值采样(max pooling)两种形式。
卷积神经网络分为了三部分,第一部分为输入层,第二部分由若干个卷积层和池化层 组成,第三部分为一个全连接的多层感知分类器构成。
卷积神经网络
Convolutional layer :卷积层
Pooling layer :池化层
Fully connected layer: 全连接层
卷积层工作原理

卷积层工作原理
卷积层的作用就是把数据通过weight变成特征向量。
池化层工作原理
池化层的作用就是把数据用最大值或者平均值代替。达到降低数据量的目的。
## **4. Python 代码实现(使用TensorFlow)**
## **代码片段**
1. tensorflow 的卷积层
```python
tf.nn.conv2d(input,#输入的应该是一个四维图像数据[batch,in_height,in_width,in_channels]
filter, #卷积核的四维数据[height,width,in_channels,out_channels]
trides,
padding,
use_cudnn_on_gpu=None,
data_format=None,#表示输入的格式默认为NHWC
name=None)
```
\2. tensorflow 的池化层
```python
tf.nn.max_pool(value,#输入的是一个四维数据[batch,height,width,channels]
ksize,# 池化窗口大小[1,height,width,1]
strides,
padding,
data_format=’NHWC’,
name=None)
```
## **环境安装**
1.安装tensorflow
```text
pip install tensorflow 或者 pip install tensorflow-gpu
```
\2. 安装PIL
> 推荐使用whl进行安装 :[Pillow的地址](https://link.zhihu.com/?target=https%3A//www.lfd.uci.edu/~gohlke/pythonlibs/)
\3. 安装科学计算库numpy
```text
pip install numpy
```
这一步,我们引入了所需的库,并且声明了一个changeImage的转换方法
```python
import tensorflow as tf
from tensorflow.examples.tutorials.mnist import input_data
mnist = input_data.read_data_sets("/tmp/data/", one_hot=True)
from PIL import Image
import base64
import re
import numpy as np
import time
# 创建一个changeImage方法将base64图片转化为模型可用的数据集,方便服务器之间传输
def changeImage(dataUrl):
dataUrl = re.sub('^data:image/.+;base64,','',dataUrl)
image_s = base64.b64decode(dataUrl)
fileName ='test.jpg'
file= open(fileName,'wb')
file.write(image_s)
file.close()
img = Image.open('./test.jpg').convert('L')
if img.size[0] != 28 or img.size[1] != 28:
img = img.resize((28, 28))
arr = []
for i in range(28):
for j in range(28):
pixel = 1.0 - float(img.getpixel((j, i)))/255.0
arr.append(pixel)
image_data = np.array(arr).reshape((1,784))
return image_data
```
这一步,我们声明了一些神经网络的参数
```python
learning_rate = 0.001 #学习率
batch_size = 128 #批大小
num_steps = 500 #使用的样本数量
display_step = 50 #显示间隔
num_input = 784 #image shape:28*28
num_classes = 10 # MNIST total classes (0-9 digits)
dropout = 0.75 #用于随机丢弃,防止过拟
X = tf.placeholder(tf.float32, [None, num_input])
Y = tf.placeholder(tf.float32, [None, num_classes])
keep_prob = tf.placeholder(tf.float32)
```
创建我们的模型
```python
def conv2d(x, W, b, strides=1):
# Conv2D wrapper, with bias and relu activation
x = tf.nn.conv2d(x, W, strides=[1, strides, strides, 1], padding='SAME')
x = tf.nn.bias_add(x, b)
return tf.nn.relu(x)
def maxpool2d(x, k=2):
# MaxPool2D wrapper
return tf.nn.max_pool(x, ksize=[1, k, k, 1], strides=[1, k, k, 1],
padding='SAME')
# 创建模型
def conv_net(x, weights, biases, dropout):
x = tf.reshape(x, shape=[-1, 28, 28, 1])
# Convolution Layer
conv1 = conv2d(x, weights['wc1'], biases['bc1'])
# Max Pooling (down-sampling)
conv1 = maxpool2d(conv1, k=2)
# Convolution Layer
conv2 = conv2d(conv1, weights['wc2'], biases['bc2'])
# Max Pooling (down-sampling)
conv2 = maxpool2d(conv2, k=2)
# Fully connected layer
# Reshape conv2 output to fit fully connected layer input
fc1 = tf.reshape(conv2, [-1, weights['wd1'].get_shape().as_list()[0]])
fc1 = tf.add(tf.matmul(fc1, weights['wd1']), biases['bd1'])
fc1 = tf.nn.relu(fc1)
# Apply Dropout
fc1 = tf.nn.dropout(fc1, dropout)
# Output, class prediction
out = tf.add(tf.matmul(fc1, weights['out']), biases['out'])
return out
```
设置我们的权重和偏移,损失函数,梯度下降,初始化参数,初始化模型保存
```python
# 设置权重和偏移
weights = {
# 5x5 conv, 1 input, 32 outputs
'wc1': tf.Variable(tf.random_normal([5, 5, 1, 32])),
# 5x5 conv, 32 inputs, 64 outputs
'wc2': tf.Variable(tf.random_normal([5, 5, 32, 64])),
# fully connected, 7*7*64 inputs, 1024 outputs
'wd1': tf.Variable(tf.random_normal([7*7*64, 1024])),
# 1024 inputs, 10 outputs (class prediction)
'out': tf.Variable(tf.random_normal([1024, num_classes]))
}
biases = {
'bc1': tf.Variable(tf.random_normal([32])),
'bc2': tf.Variable(tf.random_normal([64])),
'bd1': tf.Variable(tf.random_normal([1024])),
'out': tf.Variable(tf.random_normal([num_classes]))
}
# Construct model
logits = conv_net(X, weights, biases, keep_prob)
prediction = tf.nn.softmax(logits)
pred =
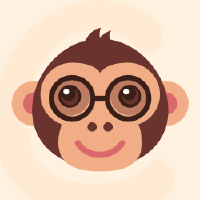
shejizuopin
- 粉丝: 1w+
- 资源: 1303
最新资源
- 【年度调薪】年度薪酬预算执行情况报告.xls
- 【年度调薪】调薪考核表.xls
- 【年度调薪】调薪矩阵表(HR总监绝密).xls
- 【年度调薪】度员工调薪登记表.xlsx
- 【年度调薪】薪资等级结构表.xls
- 【年度调薪】调薪调岗流程表格.xls
- 【年度调薪】部门年度薪资调整套级审批表.xlsx
- 【年度调薪】调薪流程.xlsx
- 【年度调薪】年度员工调薪登记表.xlsx
- 【年度调薪】员工调薪评估.xlsx
- 【年度调薪】员工加薪明细表.xlsx
- 【年度调薪】员工调薪记录表.xlsx
- 【年度调薪】HR疑难操作之调岗调薪(实务篇).doc
- 【年度调薪】工资评定调薪方案.doc
- 【年度调薪】年度调薪方案.doc
- 【年度调薪】调岗调薪操作技巧.doc
资源上传下载、课程学习等过程中有任何疑问或建议,欢迎提出宝贵意见哦~我们会及时处理!
点击此处反馈



- 1
- 2
- 3
- 4
前往页