# Imitation Learning
This repo contains simple PyTorch implementation of some Reinforcement Learning algorithms:
- Advantage Actor Critic (A2C) - a synchronous variant of [*A3C*](https://arxiv.org/abs/1602.01783)
- Proximal Policy Optimization (PPO) - one of the most popular RL algorithms [*PPO*](https://arxiv.org/abs/1707.06347),
[*Truly PPO*](https://arxiv.org/abs/1903.07940),
[*Implementation Matters*](https://arxiv.org/abs/2005.12729),
[*A Large-Scale Empirical Study of PPO*](https://arxiv.org/abs/2006.05990)
- On-policy Maximum A Posteriori Policy Optimization (V-MPO) - Algorithm that DeepMind used in their last works [*V-MPO*](https://arxiv.org/abs/1909.12238) (not working yet...)
- Behavior Cloning (BC) - simple technique to clone some expert behaviour into new policy
Each algorithm supports vector/image/dict observation spaces and discrete/continuous action spaces.
## Why repo is called "Imitation Learning"?
When I started this project and repo, I thought that Imitation Learning would be my main focus,
and model-free methods would be used only at the beginning to train 'experts'.
However, it appeared that PPO implementation (and its tricks) took more time than I expected.
As the result now most of the code is related to PPO, but I am still interested in Imitation Learning and going to add several related algorithms.
## Current Functionality
For now this repo contains some model-free on-policy algorithm implementations: A2C, PPO, V-MPO and BC.
Each algorithm supports discrete (Categorical, Bernoulli, GumbelSoftmax) and continuous (Beta, Normal, tanh(Normal)) policy distributions,
and vector or image observation environments. Beta and tanh(Normal) works best in my experiments (tested on BipedalWalker and Humanoid environments).
As found in paper [*Implementation Matters*](https://arxiv.org/abs/2005.12729),
PPO algo works mostly because of "code-level" optimizations. Here I implemented most of them:
- [x] Value function clipping (works better without it)
- [x] Observation normalization & clipping
- [x] Reward normalization/scaling & clipping
- [x] Orthogonal initialization of neural network weights
- [x] Gradient clipping
- [ ] Learning rate annealing (will be added)
In addition, I implemented roll-back loss from [*Truly PPO paper*](https://arxiv.org/abs/1903.07940), which works very well,
and 'advantage-recompute' option from [*A Large-Scale Empirical Study of PPO paper*](https://arxiv.org/abs/2006.05990).
For image-observation environments I added special regularization similar to [*CURL paper*](https://arxiv.org/abs/2004.04136),
but instead of contrastive loss between features from convolutional feature extractor,
I directly minimize D_KL between policies on augmented and non-augmented images.
As for Imitation Learning algorithms, there is only Behavior Cloning for now, but more will be added.
#### Code structure
.
├── algorithms
├── agents # A2C, PPO, V-MPO, BC, any different agent algo...
└── ... # all different algorithm parts: neural networks, probability distributions, etc.
├── experts # checkpoints of trained models, *.pth files with nn model and weights and *.py scripts with model definition.
├── train_scripts # folder with train sripts.
├── ppo/humanoid.py # train script for humanoid environment.
├── ... # other train configs.
└── test.py # script for testing trained agent.
├── trainers # implementation of trainers for different algorithms. Trainer is a manager that controls data-collection, model optimization and testing.
└── utils # all other 'support' functions that does not fit in any other folder.
#### Training example
Each experiment is described in config, look at examples here: [folder](train_scripts).
Example of training PPO agent on CartPole-v1 env:
```bash
python train_scripts/ppo/cart_pole.py
```
Training results (including training config, tensorboard logs and model checkpoints) will be saved in ```log_dir``` folder.
Obtained policy:

#### Testing example
Results of trained policy may be shown with ```train_scripts/test.py``` script.
This script is able to:
- just show how policy acts in environment
- measure mean reward and episode len over some number of episodes
- record demo file with trajectories
Type ```python train_scripts/test.py -h``` in the terminal to see detailed description of available arguments.
#### Behavior Cloning example
Demo file for BC is expected to be .pickle with episodes list inside.
An episode is a list of \[observations, actions, rewards\], where observations = \[obs_0, obs_1, ..., obs_T\],
same for action and rewards.
- Record demo file from trained policy:
```bash
python train_scripts/test.py -f logs/cart_pole/a2c_exp_0/ -p 10 -n 10 -d demo_files/cartpole_demo_10_ep.pickle -t -1 -r
```
- Prepare config to train BC: [config](train_scripts/bc/cart_pole_10_episodes.py)
- Run BC training script:
```bash
python train_scripts/bc/cart_pole_10_episodes.py
```
- ???
- Enjoy policy:
```bash
python train_scripts/test.py -f logs_py/cart_pole/bc_10_episodes/ -p 1
```
#### Modular neural network definition
Each agent have optional 'observation_encoder' and 'observation_normalizer' arguments.
Observation encoder is an neural network (i.e. nn.Module), it applied directly to observation, typically an image.
Observation normalizer is an running mean-variance estimator which standardize observations, it applied after encoder.
Sometimes actor-critic trains better on such zero-mean unit-variance observations or embeddings.
To train your own neural network architecture you can just import it in config,
initialize it in 'make_agent' function, and pass as 'actor_critic' argument into agent.
#### Trained environments
GIFs of some of results:
BipedalWalker-v3: mean reward ~333, 0 fails over 1000 episodes, [config](train_scripts/py_configs/bipedal.py).

Humanoid-v3: mean reward ~11.3k, 14 fails over 1000 episodes, [config](train_scripts/py_configs/humanoid.py).

Experiments with Humanoid done in mujoco v2
which have integration bug that makes environment easier. For academic purposes it is correct to use mujoco v1.5
CarRacing-v0: mean reward = 894 ± 32, 26 fails over 100 episodes
(episode is considered failed if reward < 900),
[config](train_scripts/py_configs/car_racing.py).

## Current issues
V-MPO implementation trains slower than A2C. Probably because of not optimal hyper-parameters sampling, need to investigate.
## Further plans
- Add logging where it is possible
- Add Motion Imitation [*DeepMimic paper*](https://arxiv.org/abs/1804.02717) algo
- Add self-play trainer with PPO as backbone algo
- Support recurrent policies models and training
- ...
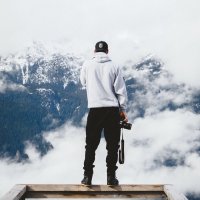
weixin_42128015
- 粉丝: 27
- 资源: 4640
最新资源
- 基于JAVA+SpringBoot+Vue+MySQL的线上买菜系统 源码+数据库(高分毕设项目).zip
- 基于JAVA+SpringBoot+Vue+MySQL的小学家校一体作业帮系统 源码+数据库(高分毕设项目).zip
- 基于JAVA+SpringBoot+Vue+MySQL的闲一品商城系统 源码+数据库+论文(高分毕设项目).zip
- LabVIEW调用基恩士XGX8500相机实现低成本画面嵌入方案,源程序开放,替代昂贵HX软件授权,LabVIEW调用 基恩士 XGX8500相机实现画面嵌入 源程序开放,方法开放 基恩士软件HX
- 基于JAVA+SpringBoot+Vue+MySQL的校园台球厅人员与设备管理系统 源码+数据库+论文(高分毕设项目).zip
- 基于JAVA+SpringBoot+Vue+MySQL的校园交友网站 源码+数据库+论文(高分毕设项目).zip
- 基于JAVA+SpringBoot+Vue+MySQL的校友社交系统 源码+数据库+论文(高分毕设项目).zip
- 二自由度悬架系统建模与振动特性深度分析:基于slx模型文件的研究与应用,1.自己写的二自由度悬架系统建模及振动特性分析模板 2.带slx模型文件 ,建模模板;二自由度悬架系统;振动特性分析;slx模型
- 基于JAVA+SpringBoot+Vue+MySQL的校园医疗保险管理系统 源码+数据库(高分毕设项目).zip
- 基于JAVA+SpringBoot+Vue+MySQL的校园志愿者管理系统 源码+数据库+论文(高分毕设项目).zip
- 基于S7-200 PLC和组态王的传送带系统设计方案与梯形图编程实战教学,基于S7-200 PLC和组态王的装车送料3传送带 带解释的梯形图程序,接线图原理图图纸,io分配,组态画面 ,基于S7-20
- 基于JAVA+SpringBoot+Vue+MySQL的校园疫情防控系统 源码+数据库+论文(高分毕设项目).zip
- 基于JAVA+SpringBoot+Vue+MySQL的学生考勤管理系统 源码+数据库+论文(高分毕设项目).zip
- 基于JAVA+SpringBoot+Vue+MySQL的协同过滤算法商品推荐系统 源码+数据库(高分毕设项目).zip
- 信捷PLC与HMI源程序的冲床送料设备对齐程序:步进电机控制,自由设定X/Y轴移动,精准计算送料步数,冲床对齐送料设备程序,包含信捷PLC以及信捷HMI源程序,程序已包含注释 PLC型号XC3-32
- 基于JAVA+SpringBoot+Vue+MySQL的学生就业管理系统 源码+数据库+论文(高分毕设项目).zip
资源上传下载、课程学习等过程中有任何疑问或建议,欢迎提出宝贵意见哦~我们会及时处理!
点击此处反馈



评论1