<p align="center"><img width="25%" src="docs/logo.png"/></p>
A Python package used for simulating spiking neural networks (SNNs) on CPUs or GPUs using [PyTorch](http://pytorch.org/) `Tensor` functionality.
BindsNET is a spiking neural network simulation library geared towards the development of biologically inspired algorithms for machine learning.
This package is used as part of ongoing research on applying SNNs to machine learning (ML) and reinforcement learning (RL) problems in the [Biologically Inspired Neural & Dynamical Systems (BINDS) lab](http://binds.cs.umass.edu/).
Check out the [BindsNET examples](https://github.com/BindsNET/bindsnet/tree/master/examples) for a collection of experiments, functions for the analysis of results, plots of experiment outcomes, and more. Documentation for the package can be found [here](https://bindsnet-docs.readthedocs.io).
[](https://travis-ci.com/BindsNET/bindsnet)
[](https://bindsnet-docs.readthedocs.io/?badge=latest)
[](http://hits.dwyl.io/Hananel-Hazan/bindsnet)
[](https://gitter.im/bindsnet_/community)
## Requirements
- Python 3.6
- `requirements.txt`
## Setting things up
### Using pip
BindsNET is available through its git repository. Issue
```
pip install git+https://github.com/BindsNET/bindsnet.git
```
to get the most recent stable release. Or, to build the `bindsnet` package from source, clone the GitHub repository, change directory to the top level of this project, and issue
```
pip install .
```
Or, to install in editable mode (allows modification of package without re-installing):
```
pip install -e .
```
To install the packages necessary to interface with the [OpenAI gym RL environments library](https://github.com/openai/gym), follow their instructions for installing the packages needed to run the RL environments simulator (on Linux / MacOS).
### Using Docker
[Link](https://hub.docker.com/r/hqkhan/bindsnet/) to Docker repository.
We also provide a Dockerfile in which BindsNET and all of its dependencies come installed in. Issue
```
docker image build .
```
at the top level directory of this project to create a docker image.
To change the name of the newly built image, issue
```
docker tag <IMAGE_ID> <NEW_IMAGE_ID>
```
To run a container and get a bash terminal inside it, issue
```
docker run -it <NEW_IMAGE_ID> bash
```
## Getting started
To run a near-replication of the SNN from [this paper](https://www.frontiersin.org/articles/10.3389/fncom.2015.00099/full#), issue
```
cd examples/mnist
python eth_mnist.py
```
There are a number of optional command-line arguments which can be passed in, including `--plot` (displays useful monitoring figures), `--n_neurons [int]` (number of excitatory, inhibitory neurons simulated), `--mode ['train' | 'test']` (sets network operation to the training or testing phase), and more. Run the script with the `--help` or `-h` flag for more information.
A number of other examples are available in the `examples` directory that are meant to showcase BindsNET's functionality. Take a look, and let us know what you think!
## Running the tests
Issue the following to run the tests:
```
python -m pytest test/
```
Some tests will fail if Open AI `gym` is not installed on your machine.
## Background
The simulation of biologically plausible spiking neuron dynamics can be challenging. It is typically done by solving ordinary differential equations (ODEs) which describe said dynamics. PyTorch does not explicitly support the solution of differential equations (as opposed to [`brian2`](https://github.com/brian-team/brian2), for example), but we can convert the ODEs defining the dynamics into difference equations and solve them at regular, short intervals (a `dt` on the order of 1 millisecond) as an approximation. Of course, under the hood, packages like `brian2` are doing the same thing. Doing this in [`PyTorch`](http://pytorch.org/) is exciting for a few reasons:
1. We can use the powerful and flexible [`torch.Tensor`](http://pytorch.org/) object, a wrapper around the [`numpy.ndarray`](https://docs.scipy.org/doc/numpy-1.13.0/reference/generated/numpy.ndarray.html) which can be transferred to and from GPU devices.
2. We can avoid "reinventing the wheel" by repurposing functions from the [`torch.nn.functional`](http://pytorch.org/docs/master/nn.html#torch-nn-functional) PyTorch submodule in our SNN architectures; e.g., convolution or pooling functions.
The concept that the neuron spike ordering and their relative timing encode information is a central theme in neuroscience. [Markram et al. (1997)](http://www.caam.rice.edu/~caam415/lec_gab/g4/markram_etal98.pdf) proposed that synapses between neurons should strengthen or degrade based on this relative timing, and prior to that, [Donald Hebb](https://en.wikipedia.org/wiki/Donald_O._Hebb) proposed the theory of Hebbian learning, often simply stated as "Neurons that fire together, wire together." Markram et al.'s extension of the Hebbian theory is known as spike-timing-dependent plasticity (STDP).
We are interested in applying SNNs to ML and RL problems. We use STDP to modify weights of synapses connecting pairs or populations of neurons in SNNs. In the context of ML, we want to learn a setting of synapse weights which will generate data-dependent spiking activity in SNNs. This activity will allow us to subsequently perform some ML task of interest; e.g., discriminating or clustering input data. In the context of RL, we may think of the spiking neural network as an RL agent, whose spiking activity may be converted into actions in an environment's action space.
We have provided some simple starter scripts for doing unsupervised learning (learning a fully-connected or convolutional representation via STDP), supervised learning (clamping output neurons to desired spiking behavior depending on data labels), and reinforcement learning (converting observations from the Atari game Space Invaders to input to an SNN, and converting network activity back to actions in the game).
## Benchmarking
We simulated a network with a population of n Poisson input neurons with firing rates (in Hertz) drawn randomly from U(0, 100), connected all-to-all with a equally-sized population of leaky integrate-and-fire (LIF) neurons, with connection weights sampled from N(0,1). We varied n systematically from 250 to 10,000 in steps of 250, and ran each simulation with every library for 1,000ms with a time resolution dt = 1.0. We tested BindsNET (with CPU and GPU computation), BRIAN2, PyNEST (the Python interface to the NEST SLI interface that runs the C++NEST core simulator), ANNarchy (with CPU and GPU computation), and BRIAN2genn (the BRIAN2 front-end to the GeNN simulator).
Several packages, including BRIAN and PyNEST, allow the setting of certain global preferences; e.g., the number of CPU threads, the number of OpenMP processes, etc. We chose these settings for our benchmark study in an attempt to maximize each library's speed, but note that BindsNET requires no setting of such options. Our approach, inheriting the computational model of PyTorch, appears to make the best use of the available hardware, and therefore makes it simple for practicioners to get the best performance from their system with the least effort.
<p align="middle">
<img src="https://github.com/Hananel-Hazan/bindsnet/blob/master/docs/BindsNET%20benchmark.png" alt="BindsNET%20Benchmark" width="503" height="403">
</p>
All simulations run on Ubuntu 16.04 LTS with Intel(R) Xeon(R) CPU E5-2687W v3 @ 3.10GHz, 128Gb RAM @ 2133MHz, and two GeForce GTX TITAN X (GM200) GPUs. Python 3.6 is used in all cases. Clock time was recorded for each simulation run.
## Citation
If you use Binds
没有合适的资源?快使用搜索试试~ 我知道了~
bindsnet:使用PyTorch模拟尖峰神经网络(SNN)

共115个文件
py:71个
rst:19个
png:7个

需积分: 45 11 下载量 181 浏览量
2021-02-04
13:35:48
上传
评论 3
收藏 23.52MB ZIP 举报
温馨提示
一个Python软件包,用于使用 Tensor功能在CPU或GPU上模拟尖峰神经网络(SNN)。 BindsNET是一个尖刺的神经网络仿真库,旨在开发用于机器学习的受生物启发的算法。 该软件包被用作正在进行的研究的一部分,该研究在中将SNN应用于机器学习(ML)和强化学习(RL)问题。 查看,以获取实验集合,结果分析功能,实验结果图等。 该软件包的文档可以在找到。 要求 Python 3.6 requirements.txt 设置东西 使用点子 BindsNET可通过其git存储库获得。 问题 pip install git+https://github.com/BindsNET/bi
资源详情
资源评论
资源推荐
收起资源包目录





































































































共 115 条
- 1
- 2
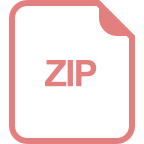
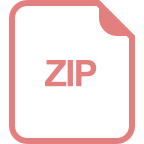
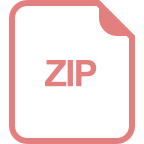
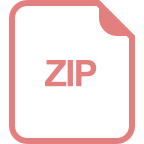
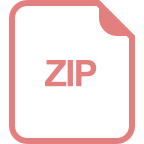
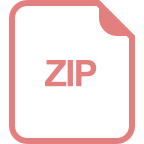
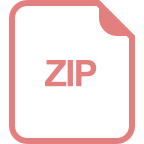
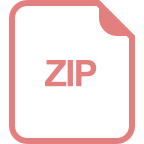
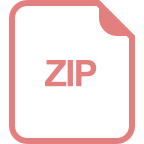
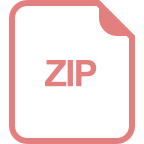
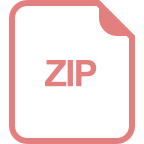
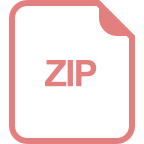
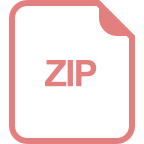
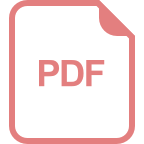
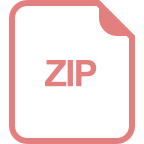
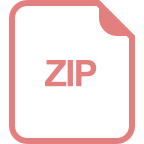
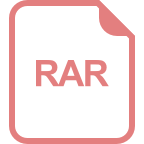
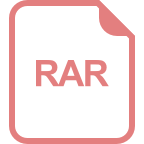
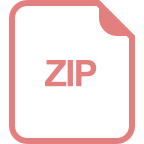
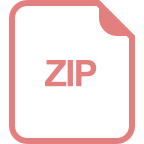
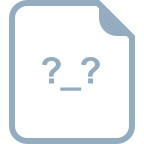
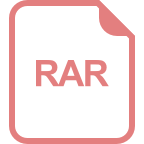
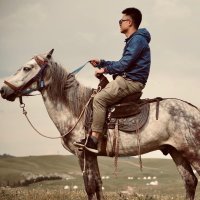
荒腔走兽
- 粉丝: 25
- 资源: 4663
上传资源 快速赚钱
我的内容管理 展开
我的资源 快来上传第一个资源
我的收益
登录查看自己的收益我的积分 登录查看自己的积分
我的C币 登录后查看C币余额
我的收藏
我的下载
下载帮助


最新资源
- 2025计算机网络技术考试题及答案.docx
- 2025驾驶员交通安全知识测试题及答案.docx
- 2025继续教育公需课必修课考试题库附含答案.docx
- 2025家政服务考试题及答案.docx
- 工程造价咨询企业基于绩效的体系设计.doc
- 2018年造价咨询公司绩效提成方案.doc
- 工程造价从业人员绩效考核制度.doc
- 工程造价企业绩效考核细则.doc
- 工程造价咨询项目考核评分制度(试行).doc
- 项目管理有限公司造价咨询薪酬管理办法.doc
- 造价咨询公司绩效提成方法.doc
- 造价咨询公司薪酬管理办法.doc
- 2025驾照C1证考试科目一必考考试题库带答案.docx
- 2025建筑八大员(材料员基础知识)考试题与答案.docx
- 2025检验类之临床医学检验技术(士)真题库附答案.docx
- 咨询公司薪酬管理办法.doc
资源上传下载、课程学习等过程中有任何疑问或建议,欢迎提出宝贵意见哦~我们会及时处理!
点击此处反馈



安全验证
文档复制为VIP权益,开通VIP直接复制

评论0