Machine_Learning_Yearning_V01
Table of Contents (draft) Why Machine Learning Strategy 4 ........................................................................................... How to use this book to help your team 6 ................................................................................ Prerequisites and Notation 7 .................................................................................................... Scale drives machine learning progress 8 ................................................................................ Your development and test sets 11 ............................................................................................ Your dev and test sets should come from the same distribution 13 ........................................ How large do the dev/test sets need to be? 15 .......................................................................... Establish a single-number evaluation metric for your team to optimize 16 ........................... Optimizing and satisficing metrics 18 ..................................................................................... Having a dev set and metric speeds up iterations 20 ............................................................... When to change dev/test sets and metrics 21 .......................................................................... Takeaways: Setting up development and test sets 23 .............................................................. Build your first system quickly, then iterate 25 ........................................................................ Error analysis: Look at dev set examples to evaluate ideas 26 ................................................ Evaluate multiple ideas in parallel during error analysis 28 ................................................... If you have a large dev set, split it into two subsets, only one of which you look at 30 ........... How big should the Eyeball and Blackbox dev sets be? 32 ...................................................... Takeaways: Basic error analysis 34 .......................................................................................... Bias and Variance: The two big sources of error 36 ................................................................. Examples of Bias and Variance 38 ............................................................................................ Comparing to the optimal error rate 39 ................................................................................... Addressing Bias and Variance 41 .............................................................................................. Bias vs. Variance tradeoff 42 ..................................................................................................... Techniques for reducing avoidable bias 43 .............................................................................. Techniques for reducing Variance 44 ....................................................................................... Error analysis on the training set 46 ........................................................................................ Diagnosing bias and variance: Learning curves 48 ................................................................. Plotting training error 50 .......................................................................................................... Interpreting learning curves: High bias 51 ............................................................................... Interpreting learning curves: Other cases 53 .......................................................................... Plotting learning curves 55 ....................................................................................................... Why we compare to human-level performance 58 .................................................................. How to define human-level performance 60 ........................................................................... Surpassing human-level performance 61 ................................................................................ Why train and test on different distributions 63 ...................................................................... Page!2 Machine Learning Yearning-Draft V0.5 Andrew NgWhether to use all your data 65 ................................................................................................ Whether to include inconsistent data 67 .................................................................................. Weighting data 68 .................................................................................................................... Generalizing from the training set to the dev set 69 ................................................................ Addressing Bias and Variance 71 ............................................................................................. Addressing data mismatch 72 ................................................................................................... Artificial data synthesis 73 ........................................................................................................ The Optimization Verification test 76 ...................................................................................... General form of Optimization Verification test 78 ................................................................... Reinforcement learning example 79 ......................................................................................... The rise of end-to-end learning 82 ........................................................................................... More end-to-end learning examples 84 .................................................................................. Pros and cons of end-to-end learning 86 ................................................................................ Learned sub-components 88 .................................................................................................... Directly learning rich outputs 89 .............................................................................................. Error Analysis by Parts 93 ....................................................................................................... Beyond supervised learning: What’s next? 94 ......................................................................... Building a superhero team - Get your teammates to read this 96 ........................................... Big picture 98 ............................................................................................................................ Credits 99
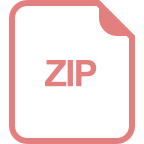
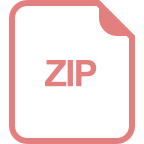
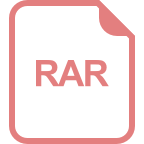
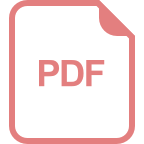
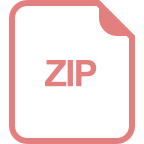
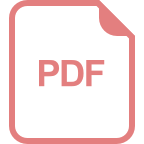
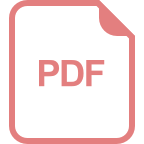
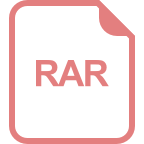




剩余22页未读,继续阅读

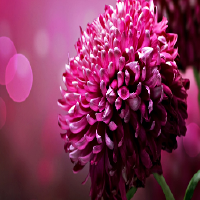
- 粉丝: 12
- 资源: 11
我的内容管理 展开
我的资源 快来上传第一个资源
我的收益
登录查看自己的收益我的积分 登录查看自己的积分
我的C币 登录后查看C币余额
我的收藏
我的下载
下载帮助


最新资源
- 2025计算机网络技术考试题及答案.docx
- 2025驾驶员交通安全知识测试题及答案.docx
- 2025继续教育公需课必修课考试题库附含答案.docx
- 2025家政服务考试题及答案.docx
- 工程造价咨询企业基于绩效的体系设计.doc
- 2018年造价咨询公司绩效提成方案.doc
- 工程造价从业人员绩效考核制度.doc
- 工程造价企业绩效考核细则.doc
- 工程造价咨询项目考核评分制度(试行).doc
- 项目管理有限公司造价咨询薪酬管理办法.doc
- 造价咨询公司绩效提成方法.doc
- 造价咨询公司薪酬管理办法.doc
- 2025驾照C1证考试科目一必考考试题库带答案.docx
- 2025建筑八大员(材料员基础知识)考试题与答案.docx
- 2025检验类之临床医学检验技术(士)真题库附答案.docx
- 咨询公司薪酬管理办法.doc


