Fictional scenario - AI online proctoring: A solution for businessA provider of proctoring technologies has made available an online proctoring system based on AI. The system is able to monitor an unlimited number of candidates in parallel, thanks to the technology used to detect exam rule violations.
The technology combines analysis of the candidate’s video and audio footage captured during the test. Video analysis includes the detection of suspicious elements or background movement (such as shadows moving behind the candidate). Body and eye movement analysis of the candidates is used to detect irregular patterns of behaviour.
Ambient sound is also analysed for the presence of suspicious sounds (e.g. keyboard chatter unsynchronised with the candidate’s movements, human speech or rustling of paper sheets). In addition to this information, the system also analyses mouse movement patterns and keystroke frequency to identify any major deviations from normal human patterns and the average candidate behaviour during the exam. Finally, the system monitors the candidate’s operating system, looking for signs of suspicious software applications, connected peripherals and sudden spikes in CPU usage. AI online proctoring: A problem for individualsWhile the system received positive feedback from institutions looking for ways to run online exams with fewer staff and facility costs, there has been much criticism towards the reliability and fairness of the system.
The competent data protection supervisory authority reacted and recommended a careful assessment of the risks raised by the use of live and automated remote proctoring with use of artificial intelligence.
Candidates complained that the system is ‘too sensitive’, constantly alerting them and distracting them from the exam due to the large number of features being monitored (e.g. body position, gaze, ambient noise, keystroke frequency). Alerts triggered by gaze analysis have often been reported to be particularly detrimental to candidates who tend to look away when thinking.
Candidates and teaching institutions have also expressed concern about the volume of data transmitted to the proctoring software provider during each examination session, claiming that such a variety of data may allow the provider to infer information about the candidate that is not necessary for the proctoring purpose. For example, socio-economic situation, level of anxiety and device fingerprint, to name but a few. |
Multimodal artificial intelligence
By Vítor Bernardo
Multimodal AI refers to artificial intelligence systems that are able to process and integrate information from multiple types of input data, such as text, images, audio and video (referred to as modalities), to produce more comprehensive and nuanced outputs. Traditional AI models typically focus on a single modality, such as text-based natural language processing (NLP)[i] or image recognition. In contrast, multimodal AI systems combine different types of data to enable more sophisticated and versatile interactions.
The human brain is inherently multimodal, seamlessly integrating information from multiple senses to form a coherent understanding of the world. Multimodal AI aims to replicate this ability, enabling machines to interpret and respond more effectively to complex real-world scenarios. For example, a multimodal AI system in a smart home could process spoken commands (audio), recognise the user's face (image) and understand contextual cues from their text messages, resulting in a more intuitive and responsive experience.
The core capability of a multimodal AI system is its ability to 'fuse' data, leveraging the strengths of each modality to gain a richer understanding. This 'fusion' can take place at different stages: sometimes raw data from different sources is combined directly, allowing the system to identify patterns across modalities, while in other cases each type of data is processed separately by specialised AI models and the results are then integrated.
One of the key advances in multimodal AI has been the development of models that can learn and process different types of data simultaneously. Transformer architectures have been particularly influential in this area, allowing models to use extensive pre-training on different datasets to build representations that bridge different modalities.
Applications of multimodal AI span several domains. In healthcare, these systems can analyse medical images alongside patient records and doctors' notes, leading to more accurate diagnoses and personalised treatments. In autonomous driving, multimodal AI combines data from cameras, LiDAR sensors and GPS to safely navigate complex environments. In entertainment, AI can create more immersive experiences by synchronising visual, audio and textual content. It can also significantly improve customer service by enabling chatbots to understand not only the user's query, but also the emotions conveyed through their voice.
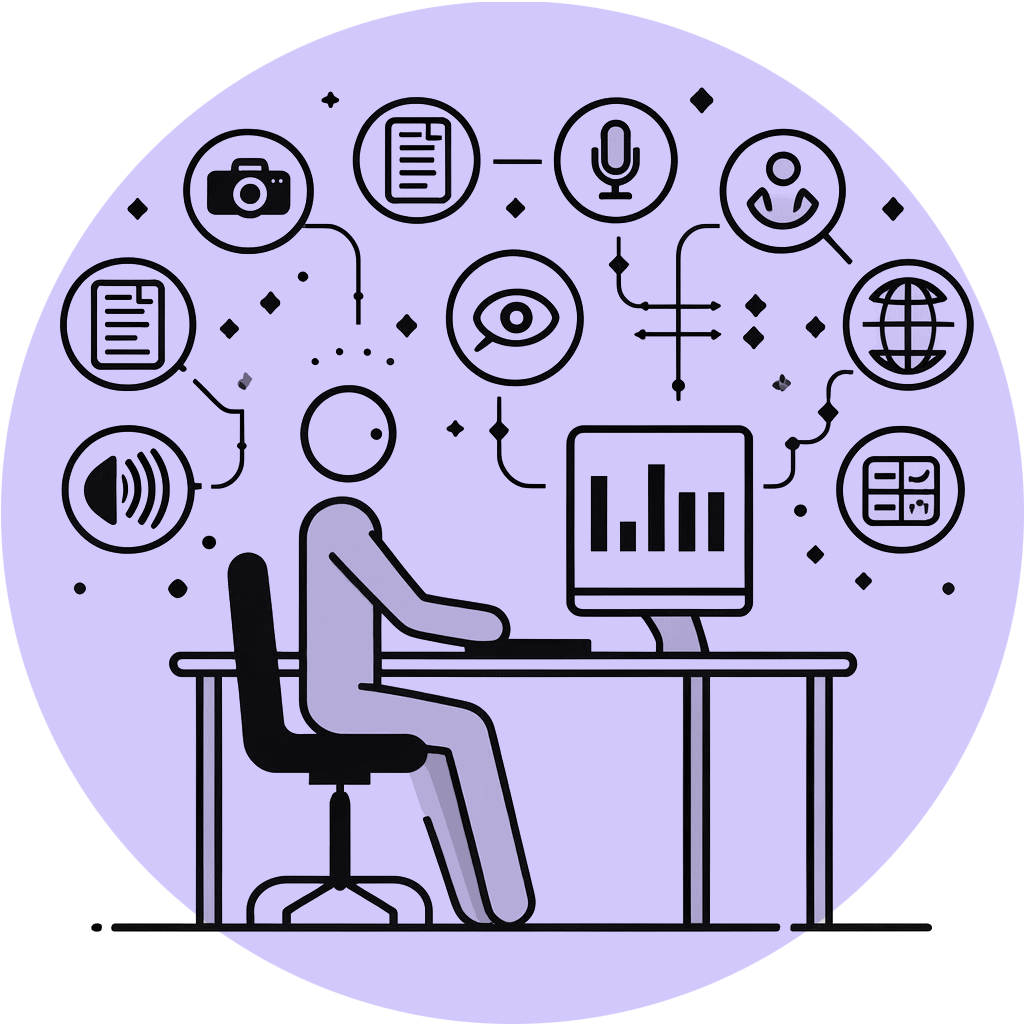
The integration of multiple modalities can also increase the robustness and reliability of AI systems. By drawing on different sources of data, these systems can compensate for the limitations or inaccuracies of individual modalities. For example, a surveillance system that uses both video and audio inputs can detect unusual activity more accurately than if it relied on a single modality.
Despite its promising potential, multimodal AI faces significant challenges. These models are typically more complex than unimodal models, requiring significant computational resources and longer training times. Integrating and synchronising different types of data is inherently complex, as each modality has its own structure, format and processing requirements, making effective combination difficult.
In addition, high-quality labelled datasets that include multiple modalities are often scarce, and collecting and annotating multimodal data is time consuming and expensive. Inconsistent data quality across modalities can also affect the performance of multimodal systems.
Interoperability between different systems and formats remains a significant technical barrier.
Development status
Systems such as GPT-4 (developed by OpenAI) and Gemini (developed by Google) are examples of existing multimodal AI models that combine text with images and video. These models can interpret visual elements, create descriptions based on images, and generate images from detailed text descriptions.
AI-enabled smart glasses with built-in cameras are another example of a new type of multimodal product, allowing the wearer to request audio and text descriptions of the images captured by the camera or to request text translations.
Early commercial applications of multimodal AI are emerging in industries like healthcare and autonomous driving, where diverse data types are combined to enhance decision-making. While impressive progress has been made, especially in handling text and images, the integration of more complex modalities and real-time processing is still being refined, meaning widespread deployment is just beginning.
It is important to note that multimodal AI is a precursor to further potential developments.
There is growing interest in making AI multi-sensory by integrating modalities such as audio, video, and 3D data to create more engaging user experiences. In home entertainment and education, augmented reality (AR) and virtual reality (VR) are expected to combine with multimodal AI to create immersive environments. In robotics, multimodal AI can improve robots' ability to process different types of input, enabling them to perform more complex tasks with greater autonomy.
The integration of data from satellites, sensors and social media could improve the monitoring and management of environmental issues such as pollution and natural disasters or enhance the sustainability of smart cities.
Communication between humans and AI systems is expected to become more natural and intuitive as systems are able to collect different types of input, from natural language and gestures to visual cues. Ultimately, multimodal AI could transform the way people interact with technology.
Potential impact on individuals
One of the distinguishing features of multimodal AI is its ability to process a wide variety of data types. When dealing with personal data, this can have both positive and negative impacts on individuals.
The ability to handle different types of data from a given subject allows systems to better understand the context, leading to more accurate inferences and decisions. However, as mentioned earlier, integrating different types of modalities is challenging, and there is no guarantee that incorporating more data will lead to better judgment and accuracy. In the worst case, multimodality can contribute to conflicting perceptions, leading to greater ambiguity and reduced accuracy in models.
Multimodal AI systems are expected to achieve co-learning, meaning that models must learn from different modalities or tasks simultaneously. However, co-learning is challenging because learning from one modality can negatively affect the model's performance in other modalities, leading to increased ambiguity and reduced accuracy, with potential implications for individuals.
In most cases, multimodality also means processing a larger volume of data. For example, training multimodal AI models requires annotated data sets (e.g. metadata associated with data sets) that allow correspondence between different types of data. This may require much more extensive data processing, potentially including personal information, which may not always be justified for the purposes of the data processing.
Another important aspect to consider when processing data from all modalities is the impact on individuals, especially when some may be more intrusive (such as neurodata). This could lead to unlawful processing of personal data.
One type of multimodal AI of particular concern is multimodal emotion recognition (MER), which can identify and interpret human emotional states by combining different signals, including but not limited to text, speech and facial cues (e.g. Google Gemini). The risk of misinterpreting emotions and manipulating users (e.g. by interpreting and adapting to user behaviour in a way that may not be clear to them) can affect individuals in a number of ways, including unfair treatment, wrong decisions and restriction of human rights.
In the joint opinion 5/2021[1] issued by the European Data Protection Supervisor (EDPS) and the European Data Protection Board (EDPB), the use of AI to infer emotions of a natural person is described as ‘highly undesirable’ and recommended for prohibition.
Suggestions for further reading
- Acosta, J. N., Falcone, G. J., Rajpurkar, P., & Topol, E. J. (2022). Multimodal biomedical AI. Nature Medicine, 28(9), 1773-1784.https://www.nature.com/articles/s41591-022-01981-2
- Baltrušaitis, T., Ahuja, C., & Morency, L. P. (2018). Multimodal machine learning: A survey and taxonomy. IEEE transactions on pattern analysis and machine intelligence, 41(2), 423-443.https://ieeexplore.ieee.org/abstract/document/8269806
- Gautam, S. (2023). Bridging Multimedia Modalities: Enhanced Multimodal AI Understanding and Intelligent Agents. Proceedings of the 25th International Conference on Multimodal Interaction.https://dl.acm.org/doi/abs/10.1145/3577190.3614225
- Liang, P. P., Zadeh, A., & Morency, L. P. (2024). Foundations & trends in multimodal machine learning: Principles, challenges, and open questions. ACM Computing Surveys, 56(10), 1-42.https://dl.acm.org/doi/full/10.1145/3656580
[1] EDPB-EDPS Joint Opinion 5/2021 on the proposal for a Regulation of the European Parliament and of the Council laying down harmonised rules on artificial intelligence (Artificial Intelligence Act), https://www.edpb.europa.eu/system/files/2021-06/edpb-edps_joint_opinion_ai_regulation_en.pdf
[i] Natural Language Processing (NLP) - A field of artificial intelligence that focuses on the interaction between computers and humans through natural language. The ultimate goal of NLP is to enable computers to understand, interpret, and respond to human language in a way that is both meaningful and useful.