
Materials innovation is one of the key drivers of major technological breakthroughs. The discovery of lithium cobalt oxide in the 1980s laid the groundwork for today’s lithium-ion battery technology. It now powers modern mobile phones and electric cars, impacting the daily lives of billions of people. Materials innovation is also required for designing more efficient solar cells, cheaper batteries for grid-level energy storage, and adsorbents to recycle CO2 from atmosphere.
Finding a new material for a target application is like finding a needle in a haystack. Historically, this task has been done via expensive and time-consuming experimental trial-and-error. More recently, computational screening of large materials databases has allowed researchers to speed up this process. Nonetheless, finding the few materials with the desired properties still requires the screening of millions of candidates.
Today, in a paper published in Nature (opens in new tab), we share MatterGen, a generative AI tool that tackles materials discovery from a different angle. Instead of screening the candidates, it directly generates novel materials given prompts of the design requirements for an application. It can generate materials with desired chemistry, mechanical, electronic, or magnetic properties, as well as combinations of different constraints. MatterGen enables a new paradigm of generative AI-assisted materials design that allows for efficient exploration of materials, going beyond the limited set of known ones.
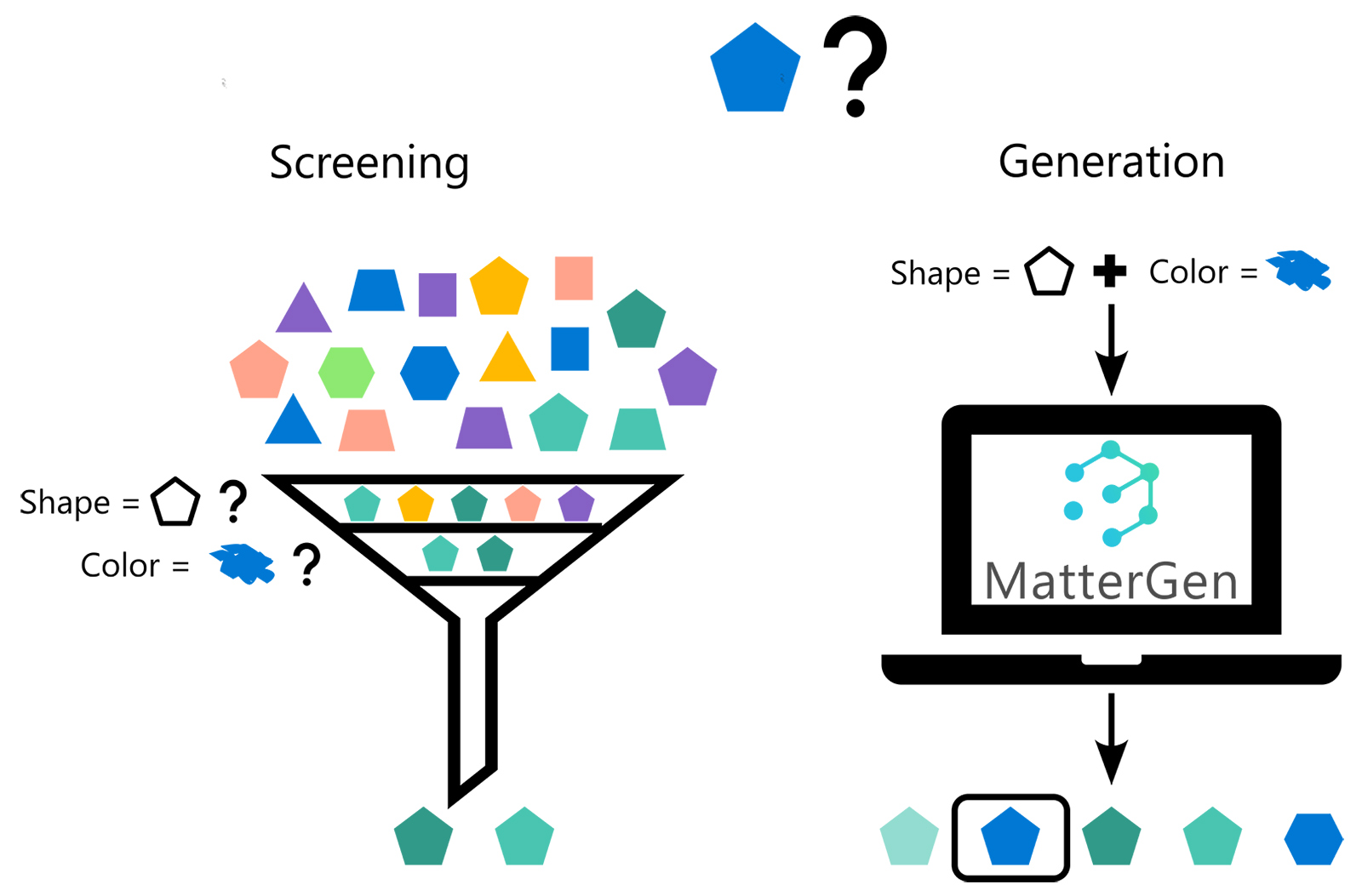
A novel diffusion architecture
MatterGen is a diffusion model that operates on the 3D geometry of materials. Much like an image diffusion model generates pictures from a text prompt by modifying the color of pixels from a noisy image, MatterGen generates proposed structures by adjusting the positions, elements, and periodic lattice from a random structure. The diffusion architecture is specifically designed for materials to handle specialties like periodicity and 3D geometry.

The base model of MatterGen achieves state-of-the-art performance in generating novel, stable, diverse materials (Figure 3). It is trained on 608,000 stable materials from the Materials Project (opens in new tab) (MP) and Alexandria (opens in new tab) (Alex) databases. The performance improvement can be attributed to both the architecture advancements, as well as the quality and size of our training data.

MatterGen can be fine-tuned with a labelled dataset to generate novel materials given any desired conditions. We demonstrate examples of generating novel materials given a target’s chemistry and symmetry, as well as electronic, magnetic, and mechanical property constraints (Figure 2).
Outperforming screening
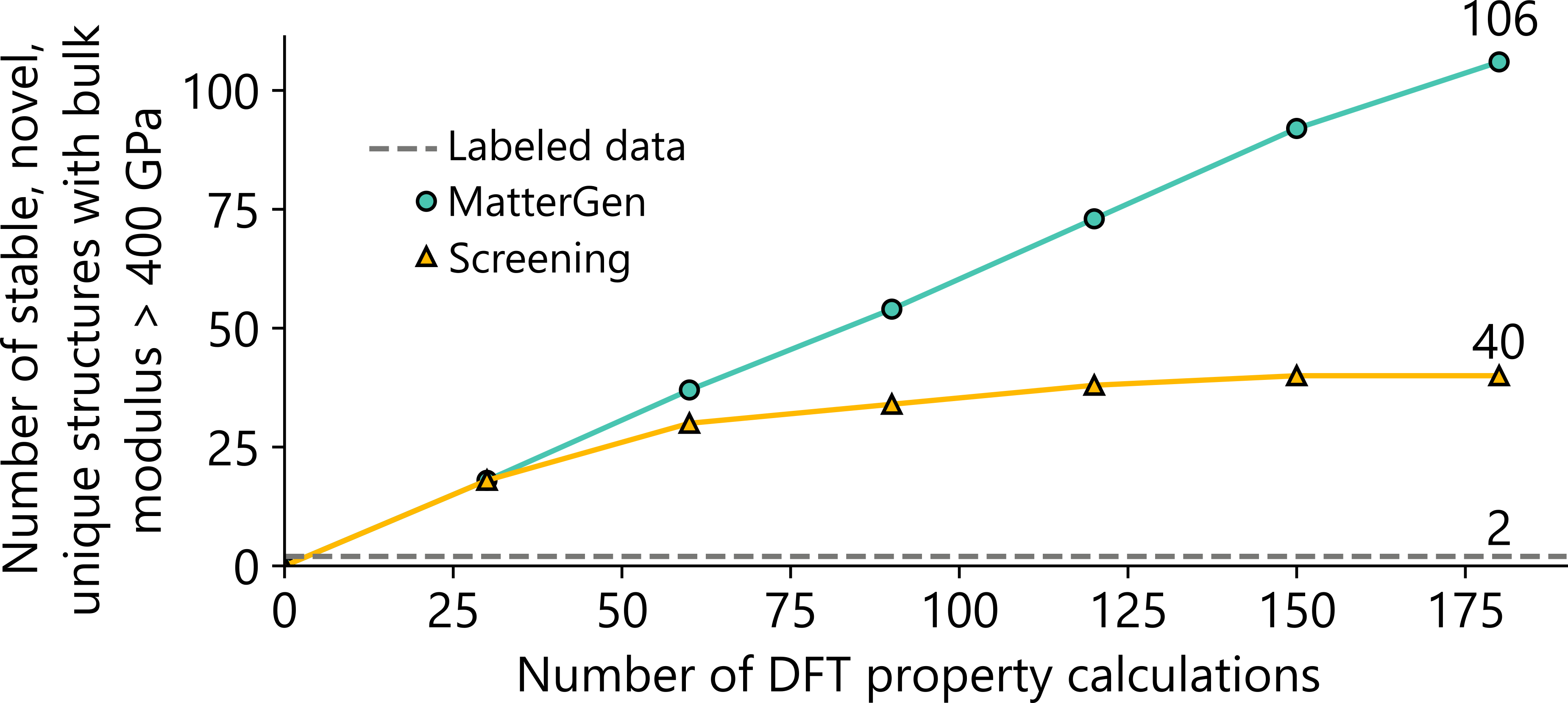
The key advantage of MatterGen over screening is its ability to access the full space of unknown materials. In Figure 4, we show that MatterGen continues to generate more novel candidate materials with high bulk modulus above 400 GPa, for example, which are hard to compress. In contrast, screening baseline saturates due to exhausting known candidates.
Spotlight: Blog post
Handling compositional disorder

Compositional disorder (Figure 5) is a commonly observed phenomenon where different atoms can randomly swap their crystallographic sites in a synthesized material. Recently (opens in new tab), the community has been exploring what it means for a material to be novel in the context of computationally designed materials, as widely employed algorithms will not distinguish between pairs of structures where the only difference is a permutation of similar elements in their respective sites.
We provide an initial solution to this issue by introducing a new structure matching algorithm that considers compositional disorder. The algorithm assesses whether a pair of structures can be identified as ordered approximations of the same underlying compositionally disordered structure. This provides a new definition of novelty and uniqueness, which we adopt in our computational evaluation metrics. We also make our algorithm publicly available (opens in new tab) as part of our evaluation package.
Experimental lab verification
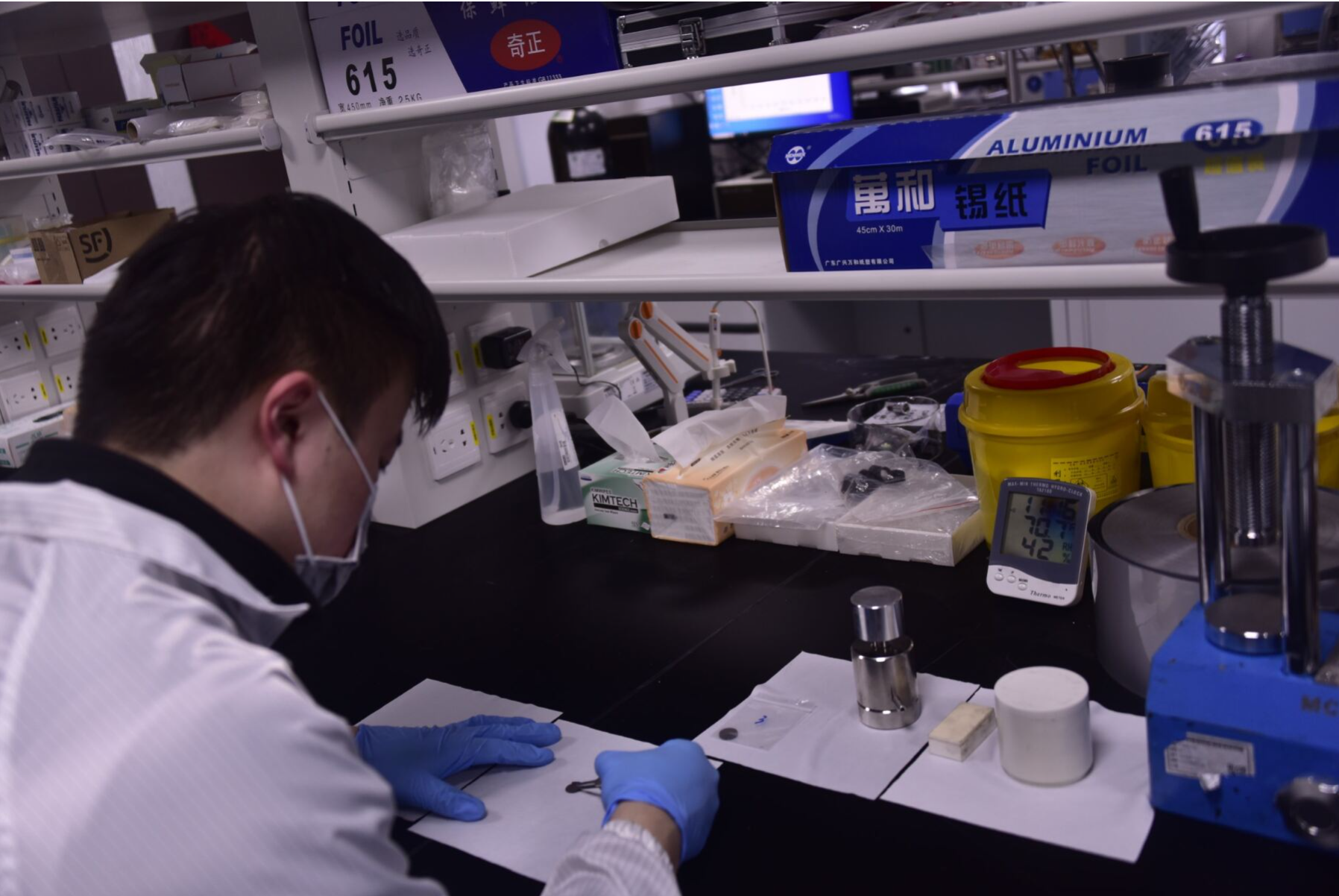
In addition to our extensive computational evaluation, we have validated MatterGen’s capabilities through experimental synthesis. In collaboration with the team led by Prof Li Wenjie from the Shenzhen Institutes of Advanced Technology (opens in new tab) (SIAT) of the Chinese Academy of Sciences, we have synthesized a novel material, TaCr2O6, whose structure was generated by MatterGen after conditioning the model on a bulk modulus value of 200 GPa. The synthesized material’s structure aligns with the one proposed by MatterGen, with the caveat of compositional disorder between Ta and Cr. Additionally, we experimentally measure a bulk modulus of 169 GPa against the 200 GPa given as design specification, with a relative error below 20%, very close from an experimental perspective. If similar results can be translated to other domains, it will have a profound impact on the design of batteries, fuel cells, and more.
AI emulator and generator flywheel
MatterGen presents a new opportunity for AI accelerated materials design, complementing our AI emulator MatterSim. MatterSim follows the fifth paradigm of scientific discovery, significantly accelerating the speed of material properties’ simulations. MatterGen in turn accelerates the speed of exploring new material candidates with property guided generation. MatterGen and MatterSim can work together as a flywheel to speed up both the simulation and exploration of novel materials.
Making MatterGen available
We believe the best way to make an impact in materials design is to make our model available to the public. We release the source code of MatterGen (opens in new tab) under the MIT license, together with the training and fine-tuning data. We welcome the community to use and build on top of our model.
Looking ahead
MatterGen represents a new paradigm of materials design enabled by generative AI technology. It explores a significantly larger space of materials than screening-based methods. It is also more efficient by guiding materials exploration with prompts. Similar to how generative AI has impacted drug discovery (opens in new tab), it will have profound impact on how we design materials in broad domains including batteries, magnets, and fuel cells.
We plan to continue our work with external collaborators to further develop and validate the technology. “At the Johns Hopkins University Applied Physics Laboratory (APL), we’re dedicated to the exploration of tools with the potential to advance discovery of novel, mission-enabling materials. That’s why we are interested in understanding the impact that MatterGen could have on materials discovery,” said Christopher Stiles, a computational materials scientists leading multiple materials discovery efforts at APL.
Acknowledgement
This work is the result of highly collaborative team efforts at Microsoft Research AI for Science. The full authors include: Claudio Zeni, Robert Pinsler, Daniel Zügner, Andrew Fowler, Matthew Horton, Xiang Fu, Zilong Wang, Aliaksandra Shysheya, Jonathan Crabbé, Shoko Ueda, Roberto Sordillo, Lixin Sun, Jake Smith, Bichlien Nguyen, Hannes Schulz, Sarah Lewis, Chin-Wei Huang, Ziheng Lu, Yichi Zhou, Han Yang, Hongxia Hao, Jielan Li, Chunlei Yang, Wenjie Li, Ryota Tomioka, Tian Xie.